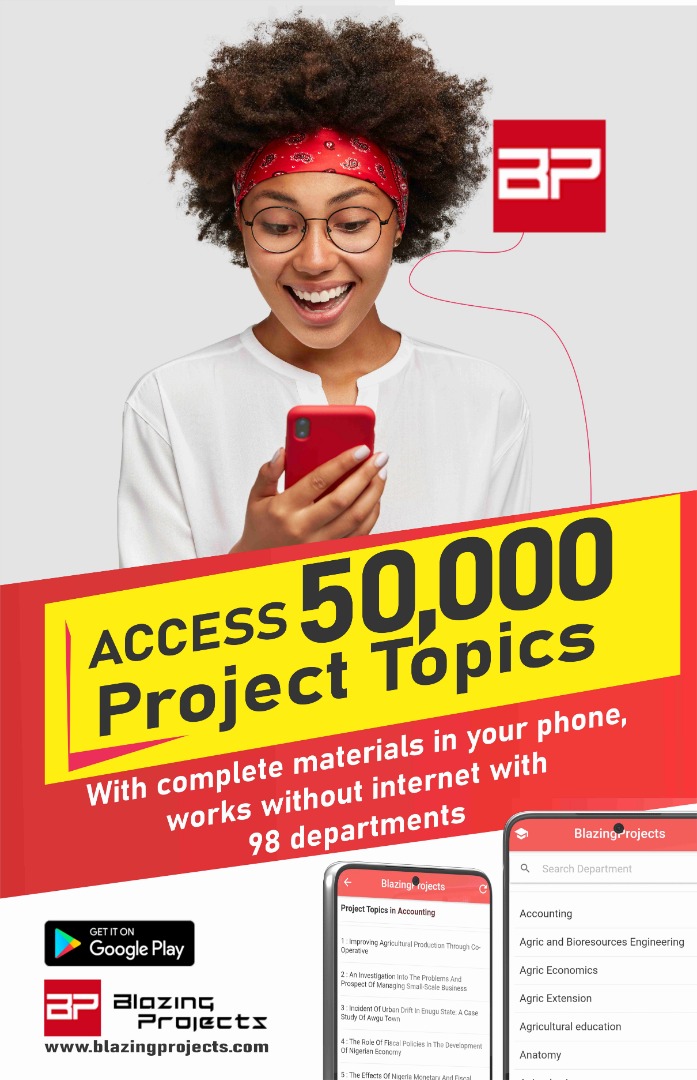
[ad_1]
Introduction
Traffic congestion is a major issue in urban areas worldwide, leading to increased travel time, fuel consumption, and pollution. Accurate prediction of traffic flow is crucial for optimizing traffic management strategies and improving overall transportation efficiency. Traditional traffic flow prediction methods rely on statistical models and data from sensors, which may not capture the complex and dynamic nature of traffic patterns.
Deep learning, a subset of artificial intelligence, has shown great promise in various fields, including image recognition, natural language processing, and speech recognition. In recent years, researchers have started exploring deep learning techniques for traffic flow prediction, leveraging the power of neural networks to analyze large volumes of data and extract meaningful patterns.
This thesis aims to investigate the effectiveness of deep learning models in predicting traffic flow and compare their performance with traditional methods. By utilizing historical traffic data, weather information, and other relevant factors, the goal is to develop accurate and reliable prediction models that can help transportation authorities make informed decisions in real-time.
Chapter 1: Introduction
1.1 Introduction
1.2 Background of study
1.3 Problem Statement
1.4 Objective of study
1.5 Limitation of study
1.6 Scope of study
1.7 Significance of study
1.8 Structure of the Thesis
1.9 Definition of terms
Chapter 2: Literature Review
2.1 Traditional traffic flow prediction methods
2.2 Deep learning in transportation research
2.3 Neural network architectures for traffic flow prediction
2.4 Data preprocessing techniques
2.5 Feature selection and engineering
2.6 Performance evaluation metrics
2.7 Hybrid models combining deep learning and traditional methods
2.8 Challenges and limitations
2.9 Future research directions
2.10 Summary of literature review
Chapter 3: Research Methodology
3.1 Data collection and preprocessing
3.2 Model selection
3.3 Hyperparameter tuning
3.4 Training and validation
3.5 Evaluation metrics
3.6 Comparison with traditional methods
3.7 Sensitivity analysis
3.8 Ethical considerations
Chapter 4: Discussion of Findings
4.1 Model performance on different datasets
4.2 Comparison with traditional methods
4.3 Impact of weather conditions on traffic flow prediction
4.4 Interpretability of deep learning models
4.5 Scalability and real-time implementation
4.6 Robustness to outliers and missing data
4.7 Practical implications for transportation management
4.8 Recommendations for future research
Chapter 5: Conclusion and Summary
5.1 Summary of findings
5.2 Contributions to the field
5.3 Implications for practice
5.4 Limitations and future research directions
Thesis Overview on Traffic Flow Prediction Using Deep Learning
Traffic congestion is a pressing issue in urban areas, leading to significant economic and environmental costs. To address this challenge, accurate traffic flow prediction is essential for optimizing traffic management strategies and improving overall transportation efficiency. Traditional prediction methods often fall short in capturing the complex and dynamic nature of traffic patterns, motivating a shift towards the use of deep learning techniques.
This thesis focuses on exploring the application of deep learning models for traffic flow prediction and comparing their performance with conventional methods. By leveraging historical traffic data, weather information, and other relevant factors, the study aims to develop robust prediction models that can provide valuable insights for transportation authorities.
Chapter 1 provides an introduction to the research topic, outlining the background, problem statement, objectives, limitations, scope, significance, and structure of the thesis. Chapter 2 reviews the existing literature on traditional traffic flow prediction methods, the role of deep learning in transportation research, neural network architectures, data preprocessing techniques, evaluation metrics, and future research directions.
In Chapter 3, the research methodology is detailed, including data collection and preprocessing, model selection, hyperparameter tuning, training, validation, and performance evaluation. Chapter 4 discusses the findings of the study, including model performance, comparison with traditional methods, impact of weather conditions, interpretability of models, scalability, and practical implications for transportation management.
Finally, Chapter 5 offers conclusions and a summary of the thesis, highlighting key findings, contributions to the field, implications for practice, limitations, and suggestions for future research. Overall, this thesis aims to advance the field of traffic flow prediction using deep learning and provide insights that can inform decision-making in transportation management.
[ad_2]
Purchase Detail
Download the complete project materials to this project with Abstract, Chapters 1 – 5, References and Appendix (Questionaire, Charts, etc), Click Here to place an order via whatsapp. Got question or enquiry; Click here to chat us up via Whatsapp.
You can also call 08111770269 or +2348059541956 to place an order or use the whatsapp button below to chat us up.
Bank details are stated below.
Bank: UBA
Account No: 1021412898
Account Name: Starnet Innovations Limited
The Blazingprojects Mobile App
Download and install the Blazingprojects Mobile App from Google Play to enjoy over 50,000 project topics and materials from 73 departments, completely offline (no internet needed) with monthly update to topics, click here to install.