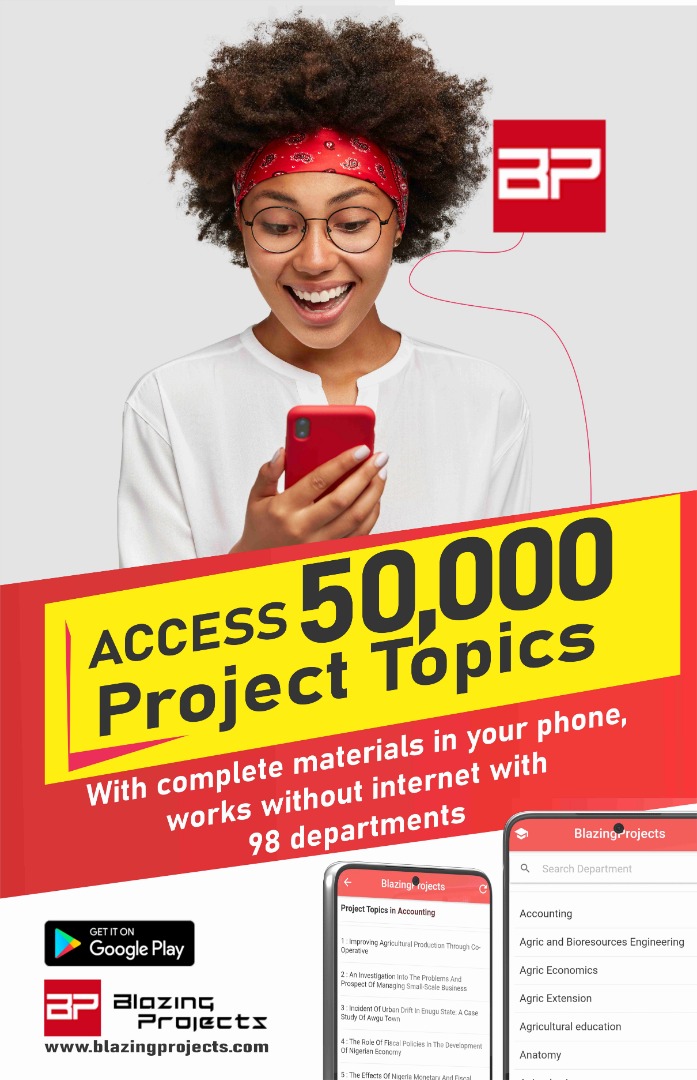
[ad_1]
Introduction
Education is a fundamental aspect of society that plays a crucial role in shaping individuals and their future. However, one of the major challenges faced in the education system is student dropout. Student dropout not only affects the individual student but also has a significant impact on the overall education system and society as a whole. In order to address this issue, there is a need for effective preventive measures that can identify potential dropouts at an early stage and provide the necessary interventions to prevent them from leaving school. Predictive modeling using educational data and machine learning has emerged as a powerful tool that can help in predicting student dropout and implementing proactive strategies for dropout prevention.
This thesis aims to investigate the use of predictive modeling for student dropout prevention using educational data and machine learning. By analyzing various factors and patterns in student data, we aim to develop predictive models that can accurately identify students at risk of dropping out. By leveraging advanced machine learning algorithms, these models can provide valuable insights for educators and policymakers to intervene early and support students in overcoming challenges that may lead to dropout.
Chapter 1: Introduction
1.1 Introduction
1.2 Background of study
1.3 Problem Statement
1.4 Objective of study
1.5 Limitation of study
1.6 Scope of study
1.7 Significance of study
1.8 Structure of the Thesis
1.9 Definition of terms
Chapter 2: Literature Review
2.1 Overview of student dropout in education
2.2 Factors contributing to student dropout
2.3 Traditional approaches to student dropout prevention
2.4 Predictive modeling in education
2.5 Machine learning techniques for predictive modeling
2.6 Previous studies on student dropout prediction
2.7 Data sources and collection methods for predictive modeling
2.8 Ethical considerations in predictive modeling for student dropout prevention
2.9 Challenges and limitations in predictive modeling for student dropout prevention
2.10 Future directions in predictive modeling for student dropout prevention
Chapter 3: Research Methodology
3.1 Research design
3.2 Data collection and preprocessing
3.3 Feature selection and engineering
3.4 Model selection and development
3.5 Model evaluation and validation
3.6 Performance metrics
3.7 Ethical considerations
3.8 Limitations of the research methodology
Chapter 4: Discussion of Findings
4.1 Analysis of predictive modeling results
4.2 Identification of key factors influencing student dropout
4.3 Comparison with traditional approaches
4.4 Implications for education policy and practice
4.5 Recommendations for future research
4.6 Limitations of the study
Chapter 5: Conclusion and Summary
5.1 Summary of key findings
5.2 Contributions to the field
5.3 Practical implications for education stakeholders
5.4 Recommendations for implementation
5.5 Conclusion
5.6 Future research directions
Thesis Overview
Student dropout is a significant issue in the education system, with far-reaching consequences for individuals and society as a whole. In recent years, there has been increasing interest in using predictive modeling and machine learning techniques to address this challenge. This thesis focuses on investigating the potential of predictive modeling for student dropout prevention using educational data and machine learning.
The introduction provides an overview of the research topic, background information, problem statement, objectives, limitations, scope, significance, and structure of the thesis. Chapter 2 presents a comprehensive literature review on student dropout, factors contributing to dropout, traditional prevention approaches, predictive modeling in education, machine learning techniques, previous studies, data sources, ethical considerations, challenges, and future directions.
Chapter 3 details the research methodology, including design, data collection, preprocessing, feature selection, model development, evaluation, validation, performance metrics, ethical considerations, and limitations. Chapter 4 discusses the findings of the study, analysis of predictive modeling results, key factors influencing dropout, comparison with traditional approaches, implications for education policy and practice, recommendations, and limitations.
Finally, Chapter 5 presents the conclusion and summary, including key findings, contributions to the field, practical implications, recommendations for implementation, conclusion, and future research directions. This thesis aims to contribute to the literature on student dropout prevention by exploring the potential of predictive modeling and machine learning in identifying at-risk students and implementing proactive strategies to support their continued education.
[ad_2]
Purchase Detail
Download the complete project materials to this project with Abstract, Chapters 1 – 5, References and Appendix (Questionaire, Charts, etc), Click Here to place an order via whatsapp. Got question or enquiry; Click here to chat us up via Whatsapp.
You can also call 08111770269 or +2348059541956 to place an order or use the whatsapp button below to chat us up.
Bank details are stated below.
Bank: UBA
Account No: 1021412898
Account Name: Starnet Innovations Limited
The Blazingprojects Mobile App
Download and install the Blazingprojects Mobile App from Google Play to enjoy over 50,000 project topics and materials from 73 departments, completely offline (no internet needed) with monthly update to topics, click here to install.