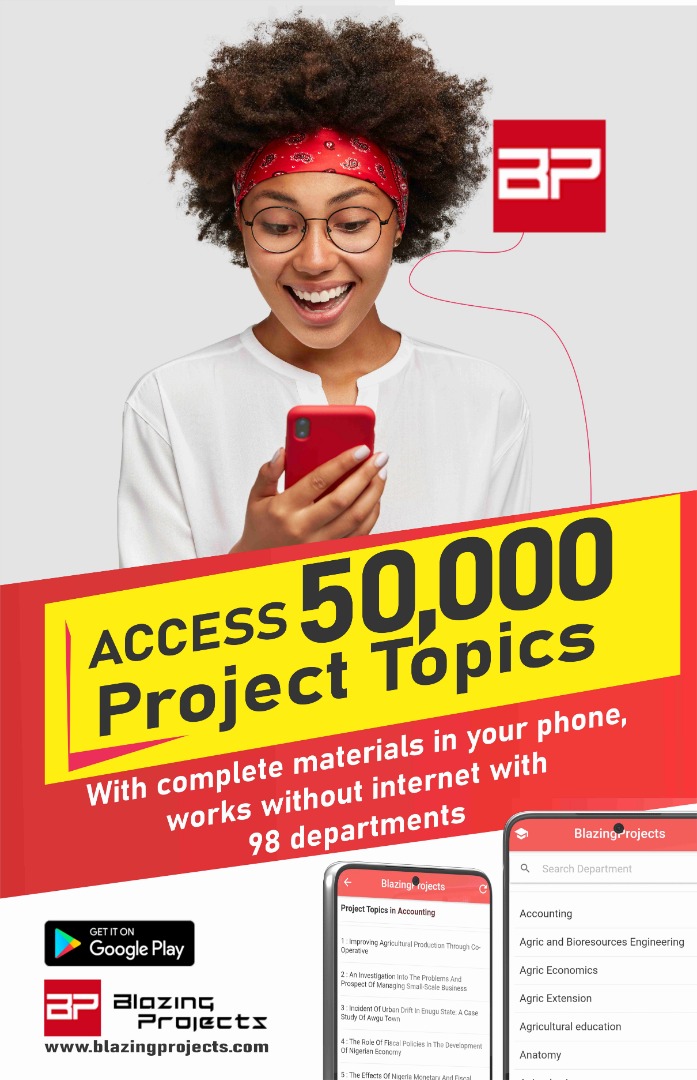
Introduction
The banking industry is becoming increasingly competitive with the rise of digital banking and fintech companies. In order to stay ahead in this competitive landscape, banks need to understand their customers better and tailor their services to meet their needs. One way to achieve this is through predictive modeling for customer lifetime value (CLV) using financial data and machine learning techniques.
Predictive modeling for CLV involves using historical data on customer behavior, transactions, and demographics to predict the future value of each customer to the bank. By accurately predicting the CLV of customers, banks can better allocate resources, personalize marketing campaigns, and improve customer retention strategies.
This thesis aims to explore the application of predictive modeling for CLV in the banking industry using financial data and machine learning. The study will examine the potential benefits and challenges of implementing predictive modeling for CLV in the banking sector, as well as provide recommendations for banks looking to leverage this technique to enhance their customer relationships.
Table of Contents
Chapter 1: Introduction
1.1 Introduction
1.2 Background of study
1.3 Problem Statement
1.4 Objective of study
1.5 Limitation of study
1.6 Scope of study
1.7 Significance of study
1.8 Structure of the Thesis
1.9 Definition of Terms
Chapter 2: Literature Review
2.1 Overview of Predictive Modeling for CLV
2.2 Importance of CLV in the Banking Industry
2.3 Previous Studies on Predictive Modeling for CLV
2.4 Machine Learning Techniques for CLV Prediction
2.5 Financial Data Analysis for CLV Prediction
2.6 Challenges in Implementing Predictive Modeling for CLV
2.7 Best Practices in CLV Prediction
2.8 Ethical Considerations in CLV Prediction
2.9 Future Trends in CLV Prediction
2.10 Summary of Literature Review
Chapter 3: Research Methodology
3.1 Research Design
3.2 Data Collection
3.3 Data Preprocessing
3.4 Model Selection
3.5 Model Training and Evaluation
3.6 Performance Metrics
3.7 Validation Techniques
3.8 Ethical Considerations
3.9 Limitations of the Study
Chapter 4: Discussion of Findings
4.1 Overview of Data Analysis
4.2 CLV Prediction Models
4.3 Performance Evaluation
4.4 Comparison of Different Machine Learning Techniques
4.5 Interpretation of Results
4.6 Implications for Banking Industry
4.7 Recommendations for Implementation
4.8 Future Research Directions
Chapter 5: Conclusion and Summary
5.1 Summary of Findings
5.2 Implications for Banking Industry
5.3 Contributions to Knowledge
5.4 Limitations of the Study
5.5 Recommendations for Future Research
5.6 Conclusion
Thesis Overview
Predictive modeling for customer lifetime value (CLV) in the banking industry using financial data and machine learning is a critical topic for banks looking to enhance their customer relationships and improve business outcomes. This thesis will explore the application of predictive modeling techniques in predicting the CLV of customers, with a focus on the banking sector.
The introduction will provide a background on the importance of CLV in the banking industry and outline the objectives, scope, and significance of the study. The literature review will summarize previous research on predictive modeling for CLV, machine learning techniques, and financial data analysis in the context of the banking industry.
The research methodology chapter will detail the research design, data collection, preprocessing, model selection, and evaluation techniques used in the study. The discussion of findings chapter will present the results of the data analysis, CLV prediction models, performance evaluation, and implications for the banking industry.
The conclusion and summary chapter will provide a summary of the findings, implications for the banking industry, contributions to knowledge, recommendations for future research, and a conclusion on the overall study. This thesis aims to provide valuable insights into the application of predictive modeling for CLV in the banking industry and offer recommendations for banks looking to implement this technique to improve their customer relationships and business outcomes.
Purchase Detail
Download the complete project materials to this project with Abstract, Chapters 1 – 5, References and Appendix (Questionaire, Charts, etc), Click Here to place an order via whatsapp. Got question or enquiry; Click here to chat us up via Whatsapp.
You can also call 08111770269 or +2348059541956 to place an order or use the whatsapp button below to chat us up.
Bank details are stated below.
Bank: UBA
Account No: 1021412898
Account Name: Starnet Innovations Limited
The Blazingprojects Mobile App
Download and install the Blazingprojects Mobile App from Google Play to enjoy over 50,000 project topics and materials from 73 departments, completely offline (no internet needed) with monthly update to topics, click here to install.