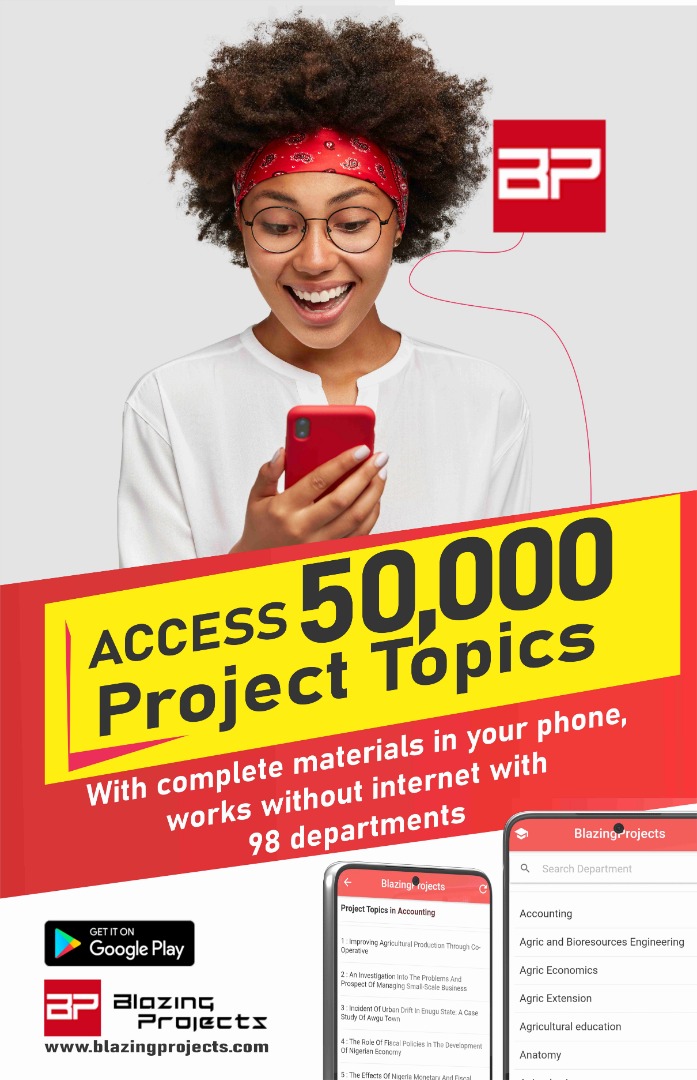
[ad_1]
Introduction
The banking industry is continuously evolving as banks seek to improve their customer acquisition strategies to remain competitive in the market. With the increase in competition, banks are turning to predictive modeling techniques to identify potential customers who are most likely to acquire their products and services. Predictive modeling, combined with credit data and machine learning algorithms, offers banks the ability to analyze historical customer data to predict future customer behaviors and preferences accurately.
Background of the Study
The availability of vast amounts of data and advances in machine learning algorithms have made predictive modeling a powerful tool for customer acquisition in the banking industry. By analyzing customer data such as credit scores, transaction history, and demographic information, banks can identify patterns and trends that can help them target the right customers with the right products at the right time. This study aims to explore the effectiveness of predictive modeling in customer acquisition within the banking industry using credit data and machine learning.
Problem Statement
Despite the potential benefits of predictive modeling for customer acquisition, many banks still struggle to effectively implement these techniques due to various challenges such as data quality issues, model complexity, and regulatory constraints. This study seeks to address these challenges and provide insights into how banks can overcome them to improve their customer acquisition strategies.
Objective of Study
The primary objective of this study is to investigate the effectiveness of predictive modeling for customer acquisition in the banking industry using credit data and machine learning. Specifically, the study aims to:
1. Explore the current trends and challenges in customer acquisition within the banking industry.
2. Evaluate the potential benefits of predictive modeling for customer acquisition.
3. Analyze the impact of using credit data and machine learning algorithms in predictive modeling for customer acquisition.
4. Provide recommendations for banks to improve their customer acquisition strategies using predictive modeling.
Limitation of Study
This study is limited to the use of credit data and machine learning algorithms for predictive modeling in customer acquisition within the banking industry. Other factors influencing customer acquisition, such as market trends, competitor strategies, and economic conditions, are not within the scope of this study.
Scope of Study
The scope of this study includes an in-depth analysis of predictive modeling techniques, credit data, and machine learning algorithms used in customer acquisition within the banking industry. The study will focus on how banks can leverage these tools to improve their customer acquisition strategies and gain a competitive advantage in the market.
Significance of Study
This study is significant as it provides valuable insights into the effectiveness of predictive modeling for customer acquisition in the banking industry. By understanding how banks can use credit data and machine learning algorithms to predict customer behavior, banks can tailor their marketing efforts and product offerings to better meet the needs of their customers.
Structure of the Thesis
This thesis is structured into five chapters. Chapter One provides an introduction to the study, including the background, problem statement, objectives, limitations, scope, significance, and structure of the thesis. Chapter Two presents a comprehensive literature review on predictive modeling, customer acquisition in the banking industry, credit data, and machine learning. Chapter Three discusses the research methodology, including data collection, analysis, and interpretation. Chapter Four presents the findings of the study and discusses their implications for customer acquisition in the banking industry. Finally, Chapter Five concludes the thesis and provides a summary of the key findings and recommendations.
Definition of Terms
1. Predictive modeling: The process of using historical data to predict future outcomes.
2. Customer acquisition: The process of attracting and gaining new customers for a business.
3. Credit data: Information about an individual’s credit history and financial behavior.
4. Machine learning: A subset of artificial intelligence that enables computers to learn from data and make predictions without being explicitly programmed.
5. Algorithms: A set of rules or instructions used to solve problems or perform tasks in a computer program.
Literature Review
1. Overview of customer acquisition strategies in the banking industry
2. Introduction to predictive modeling techniques
3. The role of credit data in customer acquisition
4. Machine learning algorithms for predictive modeling
5. Applications of predictive modeling in customer acquisition
6. Challenges of implementing predictive modeling in the banking industry
7. Comparison of traditional and predictive modeling approaches
8. Best practices for customer acquisition using predictive modeling
9. Case studies of successful customer acquisition strategies
10. Future trends in predictive modeling for customer acquisition
Research Methodology
1. Research design and approach
2. Data collection methods
3. Sampling techniques
4. Data analysis tools and techniques
5. Model development and validation
6. Ethical considerations
7. Limitations of the study
8. Research timeline and budget
Discussion of Findings
1. Analysis of predictive modeling techniques in customer acquisition
2. Impact of credit data on predictive modeling accuracy
3. Performance evaluation of machine learning algorithms
4. Comparison of different predictive modeling approaches
5. Recommendations for improving customer acquisition strategies
6. Implications for the banking industry
7. Integration of predictive modeling into existing marketing strategies
8. Future research directions
Conclusion and Summary
This chapter provides a summary of the key findings of the study, including the effectiveness of predictive modeling for customer acquisition in the banking industry using credit data and machine learning. The chapter also concludes the thesis by highlighting the implications of the study for banks and offering recommendations for future research in this field.
[ad_2]
Purchase Detail
Download the complete project materials to this project with Abstract, Chapters 1 – 5, References and Appendix (Questionaire, Charts, etc), Click Here to place an order via whatsapp. Got question or enquiry; Click here to chat us up via Whatsapp.
You can also call 08111770269 or +2348059541956 to place an order or use the whatsapp button below to chat us up.
Bank details are stated below.
Bank: UBA
Account No: 1021412898
Account Name: Starnet Innovations Limited
The Blazingprojects Mobile App
Download and install the Blazingprojects Mobile App from Google Play to enjoy over 50,000 project topics and materials from 73 departments, completely offline (no internet needed) with monthly update to topics, click here to install.