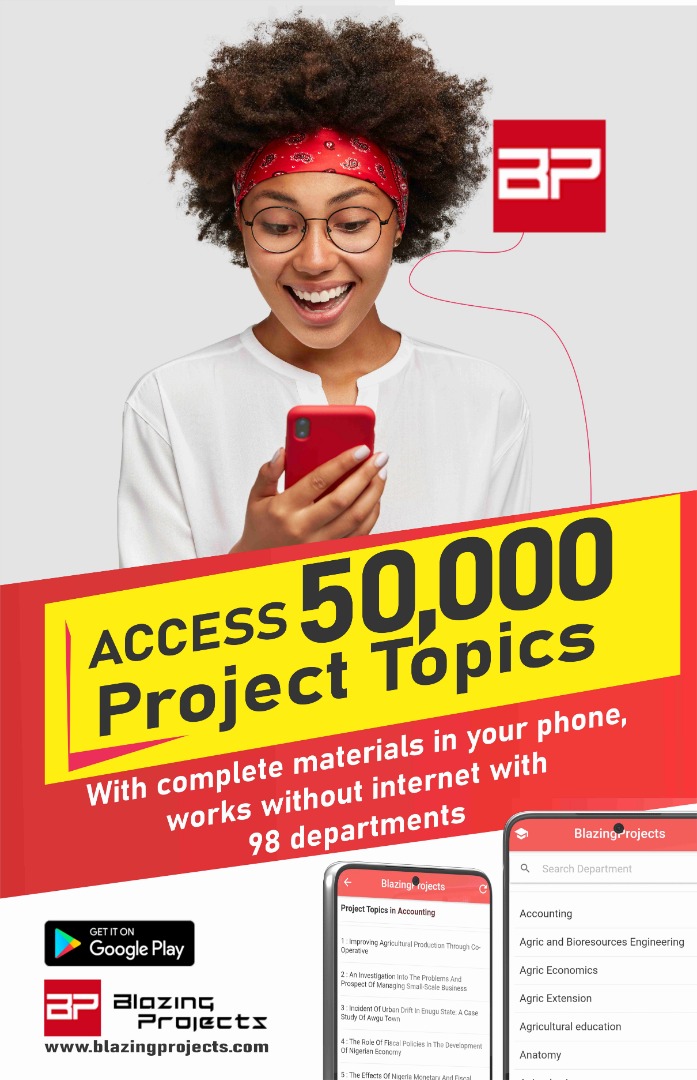
[ad_1]
Introduction:
Predictive maintenance is a proactive approach to maintenance that aims to predict when equipment failure is likely to occur, allowing for maintenance to be performed just in time to avoid downtime and costly repairs. With the advancement in sensor technology and the rise of machine learning algorithms, predictive maintenance has become more feasible and effective for manufacturing equipment. This thesis aims to explore the use of sensor data and machine learning for predictive maintenance in a manufacturing setting.
Chapter 1: Introduction
1.1 Introduction
1.2 Background of study
1.3 Problem Statement
1.4 Objective of study
1.5 Limitation of study
1.6 Scope of study
1.7 Significance of study
1.8 Structure of the Thesis
1.9 Definition of terms
Chapter 2: Literature Review
2.1 Introduction to predictive maintenance
2.2 Sensor data in manufacturing
2.3 Machine learning in predictive maintenance
2.4 Previous studies on predictive maintenance
2.5 Benefits of predictive maintenance
2.6 Challenges of predictive maintenance
2.7 Implementation of predictive maintenance
2.8 Case studies on predictive maintenance
2.9 Comparison of different predictive maintenance approaches
2.10 Future trends in predictive maintenance
Chapter 3: Research Methodology
3.1 Introduction
3.2 Research design
3.3 Data collection methods
3.4 Data analysis methods
3.5 Selection of sensors
3.6 Selection of machine learning algorithms
3.7 Model development process
3.8 Validation of predictive maintenance models
Chapter 4: Discussion of Findings
4.1 Introduction
4.2 Analysis of sensor data
4.3 Performance of machine learning algorithms
4.4 Comparison of predictive maintenance models
4.5 Impact of predictive maintenance on equipment downtime
4.6 Cost-effectiveness of predictive maintenance
4.7 Implementation challenges
4.8 Recommendations for future research
Chapter 5: Conclusion and Summary
5.1 Summary of findings
5.2 Conclusions
5.3 Implications for practice
5.4 Recommendations for further research
Thesis Overview on Predictive Maintenance for Manufacturing Equipment using Sensor Data and Machine Learning:
Predictive maintenance is a crucial aspect of ensuring the smooth operation of manufacturing equipment. This thesis is aimed at exploring the use of sensor data and machine learning algorithms for predictive maintenance in the manufacturing sector. The introduction provides a comprehensive overview of the research background, problem statement, objectives, limitations, scope, significance, structure of the thesis, and definition of terms. The literature review delves into the concepts of predictive maintenance, sensor data, machine learning, previous studies, benefits, challenges, implementation, case studies, comparison of approaches, and future trends. The research methodology section outlines the research design, data collection, data analysis, sensor and algorithm selection, model development, and validation. The discussion of findings chapter analyzes sensor data, evaluates machine learning algorithms, compares predictive maintenance models, assesses the impact on equipment downtime and cost-effectiveness, and provides implementation challenges and future research recommendations. The conclusion and summary chapter summarizes the findings, draws conclusions, discusses practical implications, and suggests areas for further research. This thesis aims to contribute to the body of knowledge on predictive maintenance for manufacturing equipment using sensor data and machine learning.
[ad_2]
Purchase Detail
Download the complete project materials to this project with Abstract, Chapters 1 – 5, References and Appendix (Questionaire, Charts, etc), Click Here to place an order via whatsapp. Got question or enquiry; Click here to chat us up via Whatsapp.
You can also call 08111770269 or +2348059541956 to place an order or use the whatsapp button below to chat us up.
Bank details are stated below.
Bank: UBA
Account No: 1021412898
Account Name: Starnet Innovations Limited
The Blazingprojects Mobile App
Download and install the Blazingprojects Mobile App from Google Play to enjoy over 50,000 project topics and materials from 73 departments, completely offline (no internet needed) with monthly update to topics, click here to install.