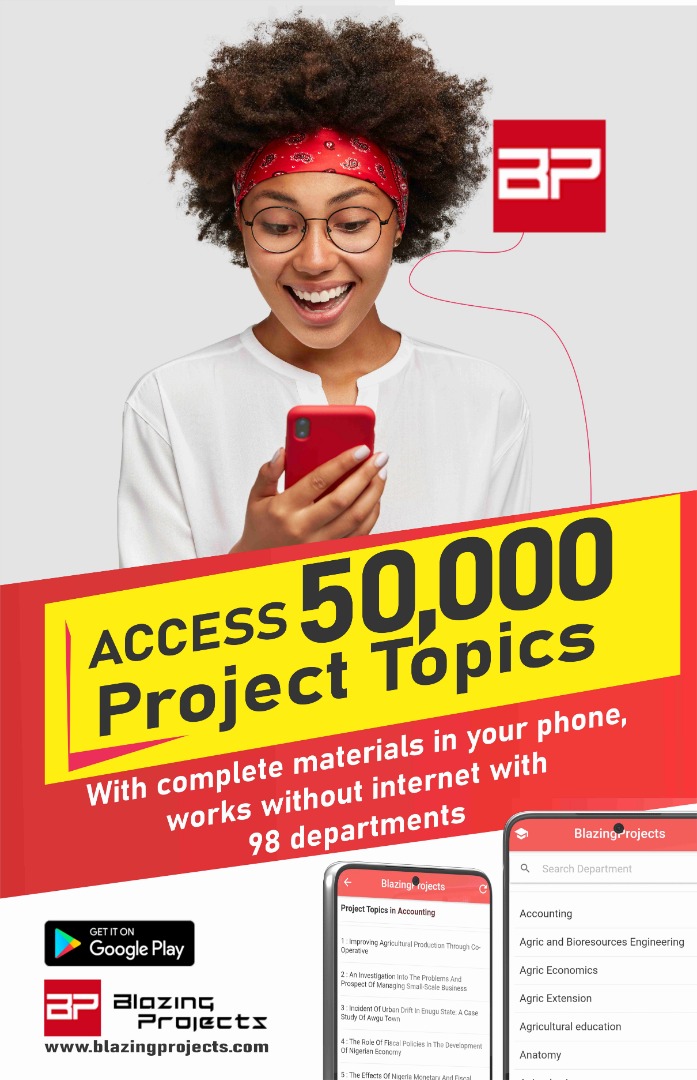
[ad_1]
Introduction
In the manufacturing industry, optimizing yield is critical for increasing profitability and competitiveness. Predictive analytics has emerged as a powerful tool for manufacturers to improve yield by identifying and addressing potential issues before they occur. This thesis explores the application of predictive analytics for manufacturing yield optimization, aiming to provide insights and recommendations for industry practitioners.
Chapter 1: Introduction
1.1 Introduction
1.2 Background of study
1.3 Problem Statement
1.4 Objective of study
1.5 Limitation of study
1.6 Scope of study
1.7 Significance of study
1.8 Structure of the Thesis
1.9 Definition of terms
Chapter 2: Literature Review
2.1 Introduction to predictive analytics in manufacturing
2.2 The importance of yield optimization in manufacturing
2.3 Key concepts and theories of predictive analytics
2.4 Previous studies on predictive analytics for yield optimization
2.5 Challenges and barriers in implementing predictive analytics in manufacturing
2.6 Best practices in predictive analytics for manufacturing yield optimization
2.7 Case studies of successful applications of predictive analytics in manufacturing
2.8 The role of advanced technologies in predictive analytics for yield optimization
2.9 Future trends and developments in predictive analytics for manufacturing
Chapter 3: Research Methodology
3.1 Research design
3.2 Data collection methods
3.3 Data analysis techniques
3.4 Sampling methods
3.5 Measurement instruments
3.6 Ethical considerations
3.7 Data validation techniques
3.8 Limitations of the study
Chapter 4: Findings and Discussion
4.1 Overview of the data analysis
4.2 Analysis of predictive analytics techniques used in manufacturing yield optimization
4.3 Comparison of different approaches to yield optimization
4.4 Interpretation of the findings
4.5 Implications for industry practitioners
4.6 Recommendations for future research
4.7 Limitations of the study
4.8 Conclusion and summary
Chapter 5: Conclusion and Summary
5.1 Summary of key findings
5.2 Contributions to the field of manufacturing yield optimization
5.3 Practical implications for industry practitioners
5.4 Recommendations for further research
5.5 Conclusion
Thesis Overview
Predictive analytics plays a crucial role in improving manufacturing yield optimization by leveraging data and advanced analytical techniques to predict outcomes and optimize processes. This thesis explores the application of predictive analytics in manufacturing yield optimization, aiming to provide insights and recommendations for industry practitioners. The introduction provides an overview of the research area, setting the context for the study. The literature review explores the existing body of knowledge on predictive analytics for yield optimization, highlighting key concepts, best practices, and case studies. The research methodology outlines the research design, data collection methods, and analysis techniques used in the study. The findings and discussion chapter presents the results of the data analysis, interpretation of findings, and implications for industry practitioners. The conclusion and summary chapter summarizes the key findings, contributions, and recommendations for future research in the field of predictive analytics for manufacturing yield optimization.
[ad_2]
Purchase Detail
Download the complete project materials to this project with Abstract, Chapters 1 – 5, References and Appendix (Questionaire, Charts, etc), Click Here to place an order via whatsapp. Got question or enquiry; Click here to chat us up via Whatsapp.
You can also call 08111770269 or +2348059541956 to place an order or use the whatsapp button below to chat us up.
Bank details are stated below.
Bank: UBA
Account No: 1021412898
Account Name: Starnet Innovations Limited
The Blazingprojects Mobile App
Download and install the Blazingprojects Mobile App from Google Play to enjoy over 50,000 project topics and materials from 73 departments, completely offline (no internet needed) with monthly update to topics, click here to install.