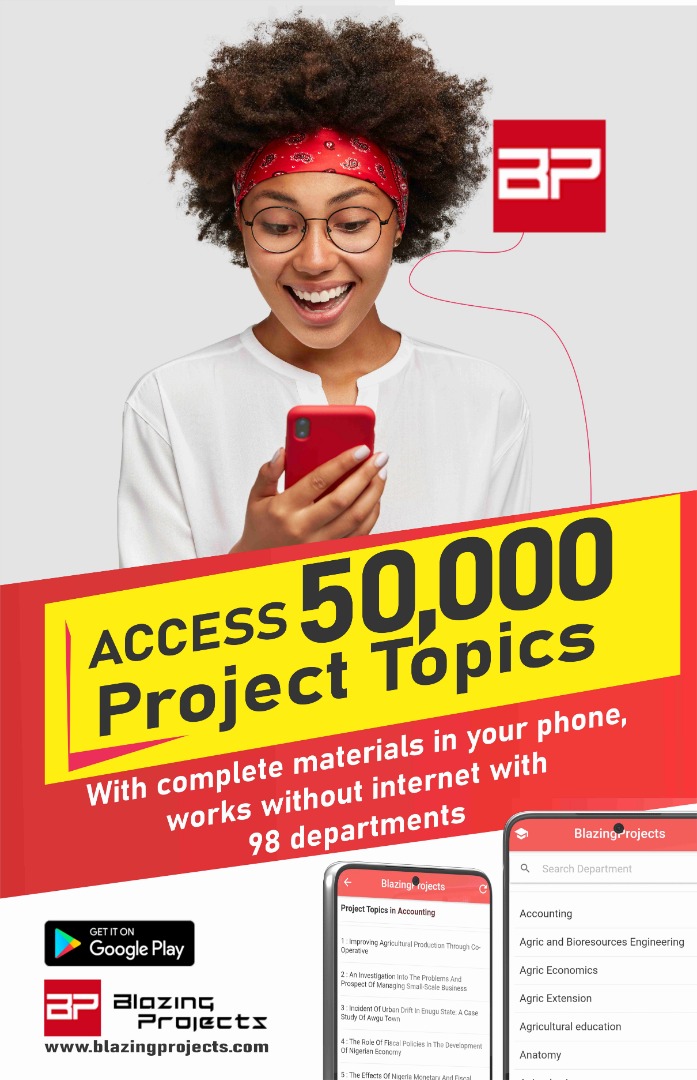
Introduction
Chronic diseases, such as heart disease, diabetes, and cancer, are major public health challenges worldwide. These diseases are responsible for a significant portion of healthcare costs and are a leading cause of mortality and morbidity. Early detection and management of chronic diseases are crucial for improving patient outcomes and reducing healthcare costs. Electronic health records (EHRs) contain a wealth of information that can be used to predict patient risk for chronic diseases. Machine learning algorithms have shown promise in analyzing EHR data to identify patients at high risk for developing chronic diseases.
This thesis aims to explore the potential of using machine learning algorithms to predict patient risk for chronic diseases using EHR data. The study will investigate the factors that contribute to the development of chronic diseases, develop predictive models using machine learning algorithms, and evaluate the performance of these models in predicting patient risk for chronic diseases.
Chapter 1: Introduction
1.1 Introduction
1.2 Background of study
1.3 Problem Statement
1.4 Objective of study
1.5 Limitation of study
1.6 Scope of study
1.7 Significance of study
1.8 Structure of the Thesis
1.9 Definition of Terms
Chapter 2: Literature Review
2.1 Overview of Chronic Diseases
2.2 Electronic Health Records
2.3 Machine Learning in Healthcare
2.4 Predictive Modeling for Chronic Diseases
2.5 Factors Contributing to Chronic Diseases
2.6 Existing Studies on Predicting Patient Risk for Chronic Diseases
2.7 Evaluation Metrics for Predictive Models
2.8 Ethical Considerations in Predictive Modeling
2.9 Challenges and Opportunities in Using Machine Learning for Predicting Chronic Diseases
2.10 Summary of Literature Review
Chapter 3: Research Methodology
3.1 Research Design
3.2 Data Collection
3.3 Data Preprocessing
3.4 Feature Selection
3.5 Model Development
3.6 Model Evaluation
3.7 Performance Metrics
3.8 Ethical Considerations
3.9 Validation of Predictive Models
Chapter 4: Discussion of Findings
4.1 Descriptive Analysis of EHR Data
4.2 Factors Contributing to Chronic Diseases
4.3 Performance of Predictive Models
4.4 Comparison of Different Machine Learning Algorithms
4.5 Interpretation of Results
4.6 Implications for Clinical Practice
4.7 Limitations of the Study
4.8 Future Research Directions
Chapter 5: Conclusion and Summary
5.1 Summary of Findings
5.2 Conclusion
5.3 Implications for Healthcare
5.4 Recommendations for Future Research
5.5 Final Thoughts
Thesis Overview
Predicting patient risk for chronic diseases using electronic health records and machine learning is a critical area of research that has the potential to revolutionize healthcare delivery. Chronic diseases are a major burden on healthcare systems globally, and early detection and management are key to improving patient outcomes and reducing healthcare costs. Electronic health records contain a wealth of information that can be leveraged to develop predictive models for identifying patients at high risk for developing chronic diseases.
This thesis aims to investigate the use of machine learning algorithms to predict patient risk for chronic diseases using EHR data. The research will involve exploring the factors contributing to the development of chronic diseases, developing predictive models using machine learning algorithms, and evaluating the performance of these models in predicting patient risk for chronic diseases. The study will also examine the ethical considerations and challenges associated with using machine learning algorithms in healthcare.
The thesis will be structured into five chapters. Chapter 1 will provide an introduction to the research topic, background information, problem statement, objectives, limitations, scope, significance, structure of the thesis, and definitions of key terms. Chapter 2 will present a comprehensive review of the literature on chronic diseases, EHRs, machine learning in healthcare, predictive modeling, and existing studies on predicting patient risk for chronic diseases. Chapter 3 will outline the research methodology, including research design, data collection, preprocessing, feature selection, model development, evaluation, performance metrics, and ethical considerations. Chapter 4 will discuss the findings of the study, including descriptive analysis of EHR data, factors contributing to chronic diseases, performance of predictive models, comparison of different machine learning algorithms, interpretation of results, implications for clinical practice, limitations, and future research directions. Chapter 5 will provide a conclusion and summary of the project, including a summary of findings, implications for healthcare, recommendations for future research, and final thoughts.
Overall, this thesis aims to contribute to the growing body of research on predicting patient risk for chronic diseases using electronic health records and machine learning. By developing accurate and reliable predictive models, healthcare providers can better identify patients at high risk for developing chronic diseases and implement timely interventions to improve patient outcomes and reduce healthcare costs.
Purchase Detail
Download the complete project materials to this project with Abstract, Chapters 1 – 5, References and Appendix (Questionaire, Charts, etc), Click Here to place an order via whatsapp. Got question or enquiry; Click here to chat us up via Whatsapp.
You can also call 08111770269 or +2348059541956 to place an order or use the whatsapp button below to chat us up.
Bank details are stated below.
Bank: UBA
Account No: 1021412898
Account Name: Starnet Innovations Limited
The Blazingprojects Mobile App
Download and install the Blazingprojects Mobile App from Google Play to enjoy over 50,000 project topics and materials from 73 departments, completely offline (no internet needed) with monthly update to topics, click here to install.