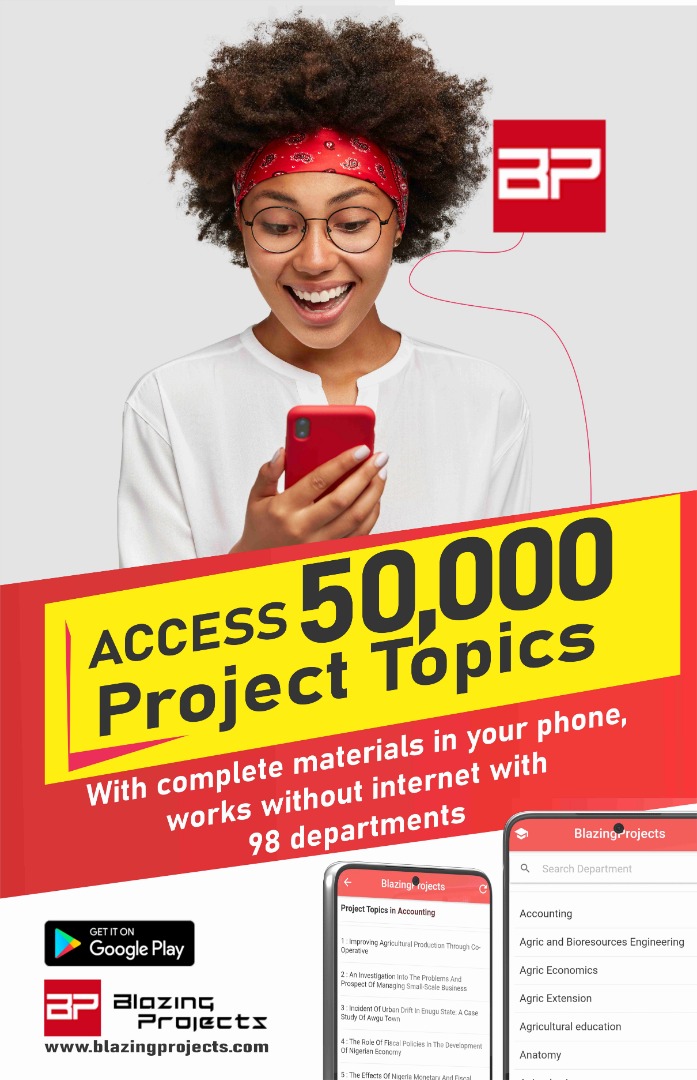
[ad_1]
Introduction
Predicting patient length of stay is an important task in healthcare management, as it can help hospitals optimize resource allocation, improve patient flow, and ultimately enhance the overall quality of care. With the increasing availability of electronic health records and advancements in machine learning techniques, there is a growing interest in developing predictive models to estimate the length of stay for individual patients at the time of hospital admission.
This thesis aims to investigate the use of hospital admission data and machine learning algorithms to predict patient length of stay. By analyzing a rich dataset of patient demographics, clinical features, and admission details, we aim to build accurate and reliable prediction models that can assist healthcare providers in planning and managing patient care effectively.
Chapter 1: Introduction
1.1 Introduction
1.2 Background of Study
1.3 Problem Statement
1.4 Objective of Study
1.5 Limitation of Study
1.6 Scope of Study
1.7 Significance of Study
1.8 Structure of the Thesis
1.9 Definition of Terms
Chapter 2: Literature Review
2.1 Introduction to Predicting patient length of stay
2.2 Machine learning in healthcare
2.3 Previous studies on patient length of stay prediction
2.4 Factors influencing patient length of stay
2.5 Data sources for patient length of stay prediction
2.6 Feature selection and engineering techniques
2.7 Evaluation metrics for predictive models
2.8 Ethical considerations in healthcare predictive analytics
2.9 Challenges in predicting patient length of stay
2.10 Future directions in patient length of stay prediction research
Chapter 3: Research Methodology
3.1 Introduction to research methodology
3.2 Data collection and preprocessing
3.3 Feature selection and engineering
3.4 Machine learning algorithms for prediction
3.5 Model training and evaluation
3.6 Optimization and tuning of predictive models
3.7 Performance metrics and evaluation criteria
3.8 Validation and generalization of models
Chapter 4: Discussion of Findings
4.1 Overview of the dataset
4.2 Descriptive statistics of patient length of stay
4.3 Performance comparison of different machine learning algorithms
4.4 Feature importance analysis
4.5 Model interpretability and explanation
4.6 Limitations and shortcomings of the predictive models
4.7 Practical implications for healthcare management
4.8 Recommendations for future research
Chapter 5: Conclusion and Summary
5.1 Summary of key findings
5.2 Contributions to the field
5.3 Implications for healthcare practice
5.4 Limitations of the study
5.5 Future research directions
Thesis Overview
The prediction of patient length of stay using hospital admission data and machine learning techniques is a critical application in healthcare management. This thesis aims to investigate the potential of predictive modeling in estimating the length of stay for individual patients at the time of hospital admission. By leveraging a rich dataset of patient demographics, clinical features, and admission details, we seek to develop accurate and reliable prediction models that can assist healthcare providers in planning and managing patient care effectively.
The thesis is organized into five chapters. Chapter 1 provides an introduction to the research topic, outlining the background, problem statement, objectives, limitations, scope, significance, structure of the thesis, and definition of terms. Chapter 2 presents a comprehensive review of the existing literature on patient length of stay prediction, machine learning in healthcare, previous studies, influencing factors, data sources, feature selection techniques, evaluation metrics, ethical considerations, challenges, and future directions.
Chapter 3 details the research methodology, including data collection and preprocessing, feature selection and engineering, machine learning algorithms, model training and evaluation, optimization, tuning, performance metrics, validation, and generalization. Chapter 4 discusses the findings from the analysis, including an overview of the dataset, descriptive statistics, performance comparison, feature importance, model interpretability, limitations, practical implications, and recommendations for future research.
Finally, Chapter 5 presents the conclusion and summary of the thesis, summarizing key findings, contributions, implications for healthcare practice, limitations, and future research directions. Through this comprehensive investigation, we aim to provide valuable insights into the application of predictive modeling in predicting patient length of stay, contributing to the advancement of healthcare management and patient care.
[ad_2]
Purchase Detail
Download the complete project materials to this project with Abstract, Chapters 1 – 5, References and Appendix (Questionaire, Charts, etc), Click Here to place an order via whatsapp. Got question or enquiry; Click here to chat us up via Whatsapp.
You can also call 08111770269 or +2348059541956 to place an order or use the whatsapp button below to chat us up.
Bank details are stated below.
Bank: UBA
Account No: 1021412898
Account Name: Starnet Innovations Limited
The Blazingprojects Mobile App
Download and install the Blazingprojects Mobile App from Google Play to enjoy over 50,000 project topics and materials from 73 departments, completely offline (no internet needed) with monthly update to topics, click here to install.