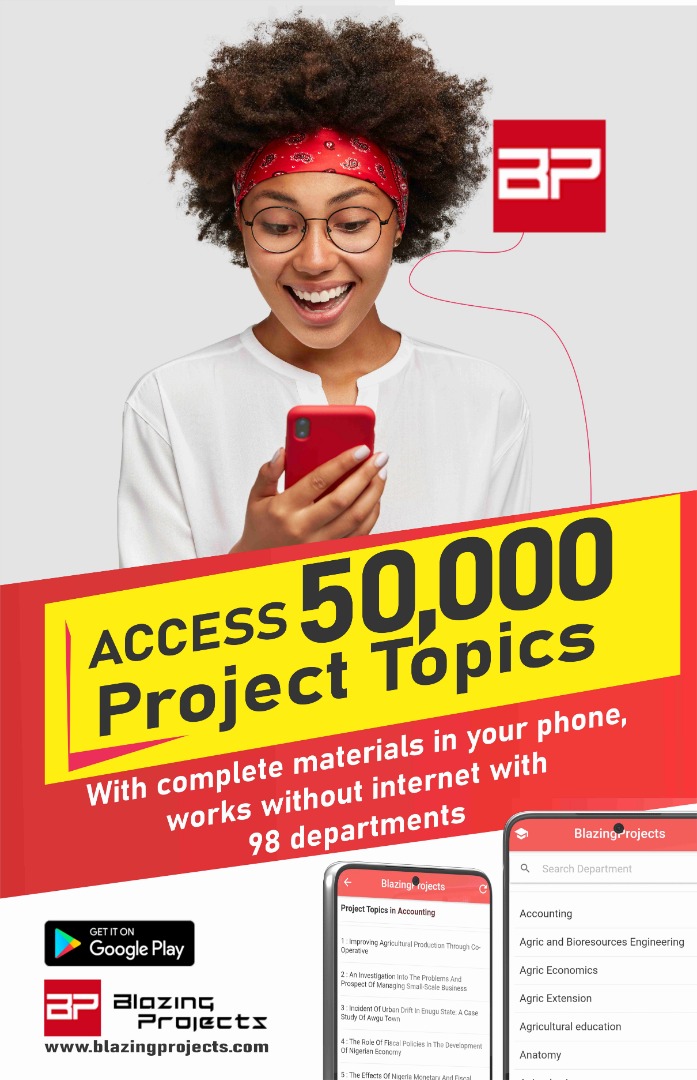
[ad_1]
Introduction
Healthcare fraud is a significant issue that affects the entire healthcare system, resulting in billions of dollars lost each year. Fraudulent activities such as billing for services not rendered, upcoding, and kickbacks not only cost the healthcare industry money but also jeopardize patient care and safety. Fraud detection in healthcare claims has become crucial in ensuring the integrity of the healthcare system and protecting patients from potential harm.
This thesis aims to explore and analyze various fraud detection techniques in healthcare claims, with a focus on improving detection accuracy and efficiency. By examining different approaches and technologies, this study seeks to identify the most effective methods for detecting and preventing healthcare fraud.
Chapter 1: Introduction
1.1 Introduction
1.2 Background of Study
1.3 Problem Statement
1.4 Objectives of Study
1.5 Limitation of Study
1.6 Scope of Study
1.7 Significance of Study
1.8 Structure of the Thesis
1.9 Definition of Terms
Chapter 2: Literature Review
2.1 Overview of Healthcare Fraud
2.2 Types of Healthcare Fraud
2.3 Current Fraud Detection Methods
2.4 Machine Learning in Fraud Detection
2.5 Data Mining Techniques
2.6 Fraud Detection Algorithms
2.7 Behavioral Analysis
2.8 Predictive Modeling
2.9 Case Studies
2.10 Summary of Literature Review
Chapter 3: Research Methodology
3.1 Research Design
3.2 Data Collection
3.3 Data Analysis
3.4 Sampling Techniques
3.5 Research Instruments
3.6 Ethical Considerations
3.7 Validity and Reliability
3.8 Limitations of Research Methodology
Chapter 4: Discussion of Findings
4.1 Analysis of Data
4.2 Comparison of Fraud Detection Techniques
4.3 Challenges and Opportunities
4.4 Recommendations for Improvement
4.5 Implications for Healthcare Industry
4.6 Future Research Directions
Chapter 5: Conclusion and Summary
5.1 Summary of Findings
5.2 Conclusions
5.3 Recommendations
5.4 Contribution to Knowledge
5.5 Limitations of the Study
5.6 Suggestions for Future Research
Thesis Overview on Fraud Detection in Healthcare Claims
Fraud detection in healthcare claims is a critical area of research that aims to address the growing issue of fraudulent activities within the healthcare system. This thesis investigates the various methods and technologies used in detecting healthcare fraud, with a specific focus on improving the accuracy and efficiency of detection processes.
The study begins with an introduction that outlines the background and significance of healthcare fraud detection, as well as the objectives and scope of the research. The literature review provides a comprehensive overview of healthcare fraud, current detection methods, and advanced technologies such as machine learning and predictive modeling.
The research methodology section describes the design and implementation of the research, including data collection, analysis, and ethical considerations. The discussion of findings analyzes the data collected and compares different fraud detection techniques, highlighting challenges and opportunities for improvement.
In the conclusion and summary chapter, key findings are summarized, conclusions are drawn, and recommendations are provided for future research and practice. This thesis aims to contribute to the knowledge base on fraud detection in healthcare claims and provide valuable insights for healthcare industry professionals, policymakers, and researchers.
[ad_2]
Purchase Detail
Download the complete project materials to this project with Abstract, Chapters 1 – 5, References and Appendix (Questionaire, Charts, etc), Click Here to place an order via whatsapp. Got question or enquiry; Click here to chat us up via Whatsapp.
You can also call 08111770269 or +2348059541956 to place an order or use the whatsapp button below to chat us up.
Bank details are stated below.
Bank: UBA
Account No: 1021412898
Account Name: Starnet Innovations Limited
The Blazingprojects Mobile App
Download and install the Blazingprojects Mobile App from Google Play to enjoy over 50,000 project topics and materials from 73 departments, completely offline (no internet needed) with monthly update to topics, click here to install.