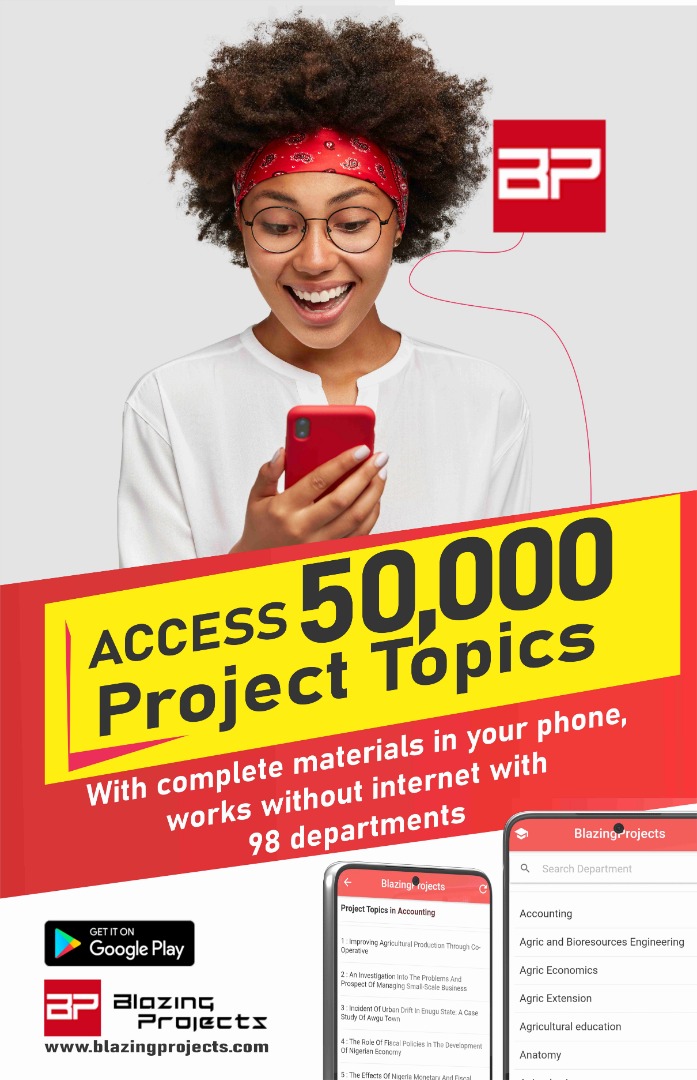
[ad_1]
Introduction
In recent years, the rapid growth of Internet of Things (IoT) devices and services has led to an increased demand for edge computing networks. Edge computing brings computation and data storage closer to the devices that generate and consume data, reducing latency and improving overall system performance. However, managing resources in edge computing networks can be challenging due to the dynamic nature of IoT devices and varying workloads.
Developing a reinforcement learning-based approach for resource allocation in edge computing networks offers a promising solution to address these challenges. Reinforcement learning is a machine learning technique that enables an agent to learn how to make decisions by interacting with its environment. By using reinforcement learning, edge computing networks can dynamically allocate resources to different tasks based on the current system state and workload conditions.
In this thesis, we will investigate the use of reinforcement learning for resource allocation in edge computing networks. We will explore different reinforcement learning algorithms, evaluate their performance in resource allocation tasks, and propose a novel approach to enhance resource allocation efficiency in edge computing networks.
Table of Contents
Chapter 1: Introduction
1.1 Introduction
1.2 Background of Study
1.3 Problem Statement
1.4 Objective of Study
1.5 Limitation of Study
1.6 Scope of Study
1.7 Significance of Study
1.8 Structure of the Thesis
1.9 Definition of Terms
Chapter 2: Literature Review
2.1 Introduction to Edge Computing Networks
2.2 Resource Allocation Techniques in Edge Computing
2.3 Reinforcement Learning in Resource Allocation
2.4 Related Work on Reinforcement Learning in Edge Computing
2.5 Challenges and Opportunities in Resource Allocation
2.6 Performance Metrics for Resource Allocation
2.7 Evaluation Methods for Resource Allocation Algorithms
2.8 Comparison of Reinforcement Learning Algorithms
2.9 State-of-the-art Techniques in Resource Allocation
2.10 Gaps in the Existing Literature
Chapter 3: Research Methodology
3.1 Research Design
3.2 Data Collection and Preprocessing
3.3 Reinforcement Learning Algorithm Selection
3.4 Experiment Setup
3.5 Evaluation Metrics
3.6 Performance Evaluation Criteria
3.7 Simulation Environment
3.8 Hypothesis Testing
3.9 Statistical Analysis
Chapter 4: Discussion of Findings
4.1 Performance of Reinforcement Learning Algorithms
4.2 Comparison with Existing Resource Allocation Techniques
4.3 Impact of System Parameters on Resource Allocation
4.4 Scalability and Robustness of the Proposed Approach
4.5 Interpretation of Results
4.6 Implications for Edge Computing Networks
4.7 Future Research Directions
4.8 Recommendations for Practitioners
Chapter 5: Conclusion and Summary
5.1 Summary of Findings
5.2 Contributions of the Thesis
5.3 Implications for Theory and Practice
5.4 Limitations of the Study
5.5 Future Research Directions
5.6 Conclusion
Thesis Overview
The increasing demand for edge computing networks to support a wide range of applications has highlighted the need for efficient resource allocation mechanisms. In this thesis, we propose a reinforcement learning-based approach to dynamically allocate resources in edge computing networks.
The literature review will examine existing resource allocation techniques in edge computing networks and evaluate the use of reinforcement learning algorithms for resource allocation tasks. The research methodology will outline the experimental setup, data collection process, and evaluation metrics used to compare the performance of reinforcement learning algorithms in resource allocation.
The discussion of findings will present the results of the experiments, including the performance of different reinforcement learning algorithms, comparison with existing techniques, and the impact of system parameters on resource allocation. The conclusion and summary chapter will summarize the key findings, contributions of the study, limitations, future research directions, and recommendations for practitioners in the field.
Overall, this thesis aims to provide insights into the potential of reinforcement learning for resource allocation in edge computing networks and contribute to the advancement of efficient resource management in IoT environments.
[ad_2]
Purchase Detail
Download the complete project materials to this project with Abstract, Chapters 1 – 5, References and Appendix (Questionaire, Charts, etc), Click Here to place an order via whatsapp. Got question or enquiry; Click here to chat us up via Whatsapp.
You can also call 08111770269 or +2348059541956 to place an order or use the whatsapp button below to chat us up.
Bank details are stated below.
Bank: UBA
Account No: 1021412898
Account Name: Starnet Innovations Limited
The Blazingprojects Mobile App
Download and install the Blazingprojects Mobile App from Google Play to enjoy over 50,000 project topics and materials from 73 departments, completely offline (no internet needed) with monthly update to topics, click here to install.