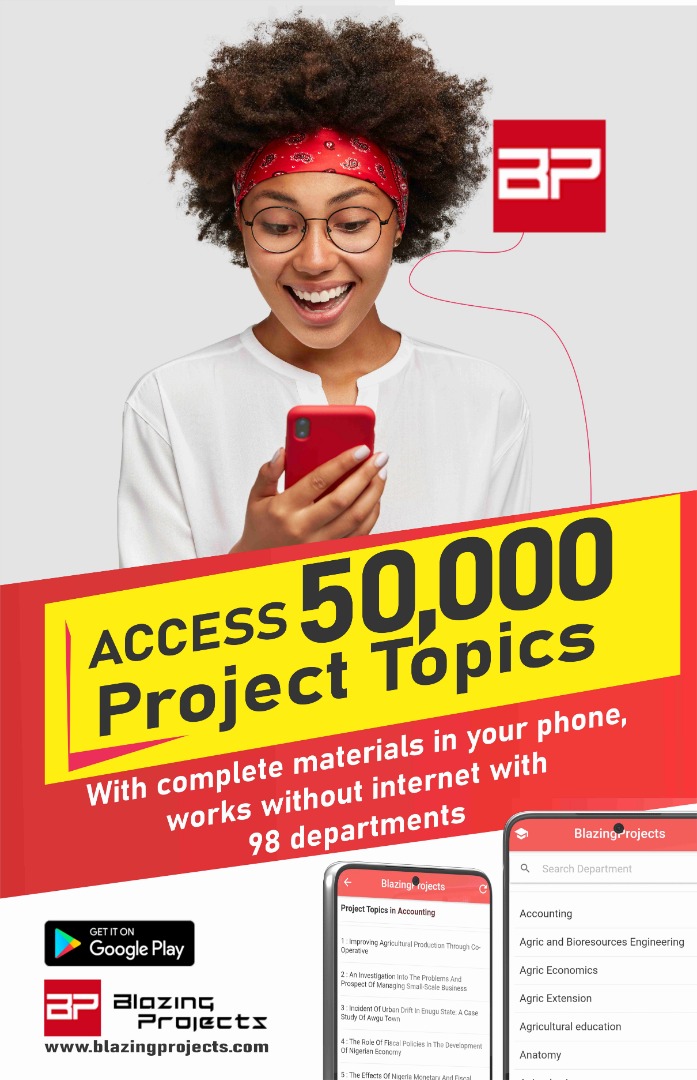
Introduction
In recent years, edge computing has emerged as a promising paradigm to address the challenges of latency, bandwidth, and reliability in traditional cloud computing systems. Edge computing enables data processing to be done closer to the source of data generation, which results in reduced latency and improved reliability for applications. However, one of the key challenges in edge computing environments is dynamic resource allocation, where resources such as computing power, storage, and network bandwidth need to be efficiently allocated to meet the varying demands of applications.
Reinforcement learning has shown great potential in addressing dynamic resource allocation problems in various domains, including edge computing. By learning from interactions with the environment, reinforcement learning agents can adaptively allocate resources to optimize the performance of applications running on edge devices. In this thesis, we propose a reinforcement learning-based approach for dynamic resource allocation in edge computing environments.
Chapter 1: Introduction
1.1 Introduction
1.2 Background of study
1.3 Problem Statement
1.4 Objective of study
1.5 Limitation of study
1.6 Scope of study
1.7 Significance of study
1.8 Structure of the Thesis
1.9 Definition of terms
Chapter 2: Literature Review
2.1 Overview of edge computing
2.2 Resource allocation in edge computing
2.3 Reinforcement learning in resource allocation
2.4 Existing approaches for dynamic resource allocation in edge computing
2.5 Challenges in dynamic resource allocation
2.6 Comparison of different reinforcement learning algorithms
2.7 Applications of reinforcement learning in edge computing
2.8 Case studies of reinforcement learning in resource allocation
2.9 Future trends in reinforcement learning for resource allocation
2.10 Summary of literature review
Chapter 3: Research Methodology
3.1 Research design
3.2 Data collection
3.3 Experimental setup
3.4 Evaluation metrics
3.5 Reinforcement learning algorithm selection
3.6 Training and testing procedures
3.7 Performance evaluation criteria
3.8 Sensitivity analysis
3.9 Ethical considerations
Chapter 4: Discussion of Findings
4.1 Analysis of experimental results
4.2 Comparison with existing approaches
4.3 Performance evaluation of reinforcement learning algorithm
4.4 Sensitivity analysis results
4.5 Implications for practice
4.6 Limitations of the study
4.7 Recommendations for future research
4.8 Conclusions drawn from findings
Chapter 5: Conclusion and Summary
5.1 Summary of key findings
5.2 Contributions of the study
5.3 Practical implications
5.4 Theoretical implications
5.5 Recommendations for practitioners
5.6 Recommendations for policy makers
5.7 Limitations of the study
5.8 Suggestions for future research
5.9 Conclusion
Thesis Overview
Developing a reinforcement learning-based approach for dynamic resource allocation in edge computing is crucial in optimizing the performance of applications running on edge devices. This thesis aims to address the challenges of dynamic resource allocation in edge computing environments by proposing a reinforcement learning-based approach. The study will begin with an introduction to the research problem, followed by a literature review on edge computing, resource allocation, and reinforcement learning.
The research methodology section will outline the experimental design, data collection methods, and evaluation metrics used to evaluate the proposed approach. The findings of the study will be discussed in detail, analyzing the experimental results, comparing them with existing approaches, and discussing the implications for practice. The conclusion and summary section will summarize the key findings, contributions, limitations, and recommendations for future research.
Overall, this thesis will contribute to the growing body of knowledge on dynamic resource allocation in edge computing and provide insights into the application of reinforcement learning in optimizing resource allocation strategies.
Purchase Detail
Download the complete project materials to this project with Abstract, Chapters 1 – 5, References and Appendix (Questionaire, Charts, etc), Click Here to place an order via whatsapp. Got question or enquiry; Click here to chat us up via Whatsapp.
You can also call 08111770269 or +2348059541956 to place an order or use the whatsapp button below to chat us up.
Bank details are stated below.
Bank: UBA
Account No: 1021412898
Account Name: Starnet Innovations Limited
The Blazingprojects Mobile App
Download and install the Blazingprojects Mobile App from Google Play to enjoy over 50,000 project topics and materials from 73 departments, completely offline (no internet needed) with monthly update to topics, click here to install.