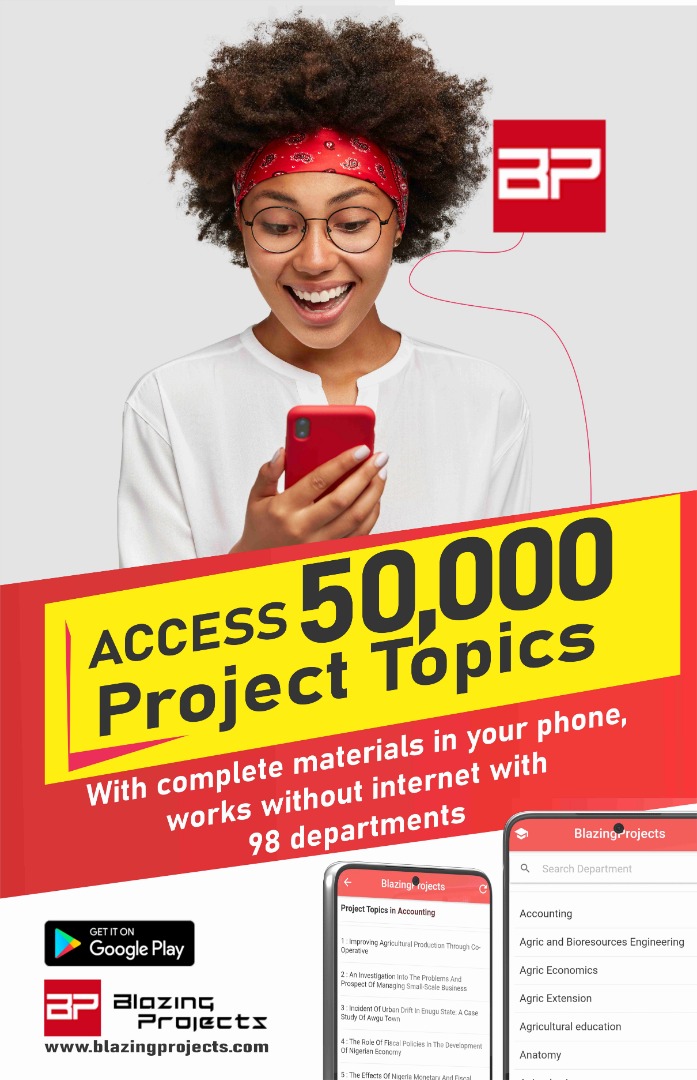
[ad_1]
Introduction
In recent years, deep learning has gained significant attention and success in various fields, including natural language processing, image recognition, and speech synthesis. One area where deep learning shows great promise is music generation and composition. Music generation involves creating new musical pieces autonomously or with minimal human intervention, while music composition involves arranging and structuring musical elements to create a coherent piece. Developing a deep learning-based system for music generation and composition has the potential to revolutionize the music industry by enabling musicians and composers to explore new creative possibilities and enhance their artistic processes.
This thesis aims to investigate the development of a deep learning-based system for music generation and composition. By leveraging the power of deep learning algorithms, such as recurrent neural networks and generative adversarial networks, we will explore how these technologies can be applied to create music that is both innovative and expressive. The system will be trained on a large dataset of musical pieces to learn the underlying patterns and structures of music, allowing it to generate new compositions that capture the essence of various musical genres and styles.
Chapter 1: Introduction
1.1 Introduction
1.2 Background of study
1.3 Problem Statement
1.4 Objective of study
1.5 Limitation of study
1.6 Scope of study
1.7 Significance of study
1.8 Structure of the Thesis
1.9 Definition of Terms
Chapter 2: Literature Review
2.1 Overview of deep learning in music generation
2.2 Traditional methods of music generation and composition
2.3 Applications of deep learning in music
2.4 Challenges and limitations of deep learning in music generation
2.5 Music representation and feature extraction
2.6 Compositional rules and musical theory
2.7 Evaluation metrics for generated music
2.8 Existing deep learning models for music generation
2.9 Comparative analysis of deep learning models
2.10 Future directions in deep learning for music generation
Chapter 3: Research Methodology
3.1 Data collection and preparation
3.2 Preprocessing and feature extraction
3.3 Model selection and architecture design
3.4 Training and evaluation procedures
3.5 Hyperparameter tuning
3.6 Performance metrics
3.7 Validation and testing
3.8 Ethical considerations
Chapter 4: Discussion of Findings
4.1 Analysis of results
4.2 Comparison with existing models
4.3 Interpretation of generated music
4.4 Musical creativity and expressiveness
4.5 Limitations and challenges
4.6 Future research directions
4.7 Implications for the music industry
4.8 Practical applications and use cases
Chapter 5: Conclusion and Summary
5.1 Summary of key findings
5.2 Contributions to the field
5.3 Limitations and future work
5.4 Final remarks
Thesis Overview
The advent of deep learning has opened up new possibilities for various fields, including music generation and composition. This thesis focuses on developing a deep learning-based system for music creation that leverages the power of recurrent neural networks and generative adversarial networks to generate novel musical compositions. By exploring the underlying patterns and structures of music, the system aims to create music that is both innovative and expressive, capturing the essence of different musical genres and styles.
In Chapter 1, we provide an introduction to the research topic, outlining the background, problem statement, objectives, limitations, scope, significance, structure of the thesis, and definition of terms. Chapter 2 presents a comprehensive literature review on deep learning in music generation, traditional methods of music creation, applications of deep learning in music, challenges and limitations, music representation, evaluation metrics, existing deep learning models, and future directions.
Chapter 3 details the research methodology, including data collection, preprocessing, feature extraction, model selection, training, evaluation, hyperparameter tuning, performance metrics, validation, testing, and ethical considerations. In Chapter 4, we discuss the findings of the research, analyzing results, comparing with existing models, interpreting generated music, discussing creativity and expressiveness, addressing limitations, suggesting future research directions, and outlining implications for the music industry.
Finally, Chapter 5 concludes the thesis by summarizing key findings, discussing contributions to the field, identifying limitations and future work, and providing final remarks. Developing a deep learning-based system for music generation and composition has the potential to revolutionize how music is created and experienced, offering new possibilities for musicians, composers, and music enthusiasts worldwide.
[ad_2]
Purchase Detail
Download the complete project materials to this project with Abstract, Chapters 1 – 5, References and Appendix (Questionaire, Charts, etc), Click Here to place an order via whatsapp. Got question or enquiry; Click here to chat us up via Whatsapp.
You can also call 08111770269 or +2348059541956 to place an order or use the whatsapp button below to chat us up.
Bank details are stated below.
Bank: UBA
Account No: 1021412898
Account Name: Starnet Innovations Limited