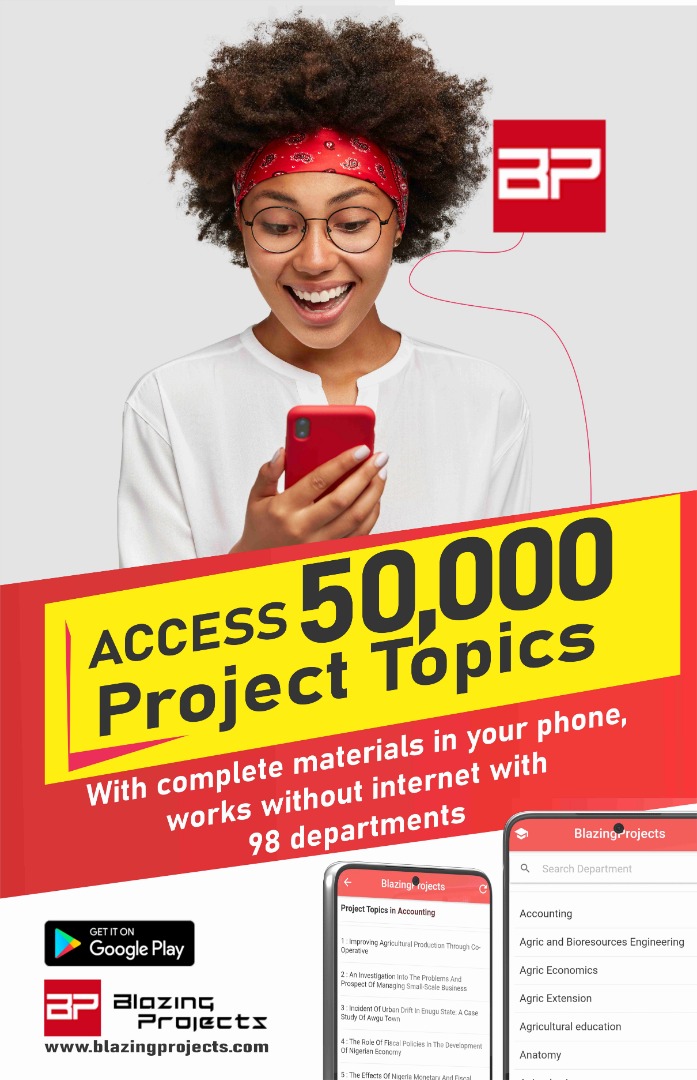
[ad_1]
Introduction:
In recent years, the explosion of data generated by businesses and organizations has necessitated the need for scalable data storage and processing solutions. Data lakes have emerged as a popular approach for storing large volumes of structured and unstructured data in a centralized repository. However, building and managing data lakes at scale pose significant challenges that require robust data engineering strategies.
This thesis aims to explore data engineering strategies for building scalable data lakes. The research will focus on addressing key challenges such as data ingestion, data quality, data governance, and data processing in the context of building and maintaining data lakes. By leveraging best practices and emerging technologies in data engineering, organizations can effectively manage and utilize their data assets for various data analytics and business intelligence applications.
Table of Contents:
Chapter 1: Introduction
1.1 Introduction
1.2 Background of study
1.3 Problem Statement
1.4 Objective of study
1.5 Limitation of study
1.6 Scope of study
1.7 Significance of study
1.8 Structure of the Thesis
1.9 Definition of Terms
Chapter 2: Literature Review
2.1 Overview of Data Lakes
2.2 Data Ingestion Strategies
2.3 Data Quality in Data Lakes
2.4 Data Governance in Data Lakes
2.5 Data Processing Technologies
2.6 Scalability in Data Lakes
2.7 Best Practices in Data Engineering
2.8 Emerging Technologies in Data Engineering
2.9 Case Studies of Successful Data Lake Implementations
2.10 Challenges and Opportunities in Data Engineering for Data Lakes
Chapter 3: Research Methodology
3.1 Research Design
3.2 Data Collection Methods
3.3 Data Analysis Techniques
3.4 Sampling Strategy
3.5 Ethical Considerations
3.6 Research Limitations
3.7 Research Validity
3.8 Research Reliability
Chapter 4: Discussion of Findings
4.1 Data Ingestion Challenges and Solutions
4.2 Data Quality Issues and Remedies
4.3 Data Governance Frameworks
4.4 Data Processing Tools and Techniques
4.5 Scalability Considerations
4.6 Implementation Best Practices
4.7 Technology Adoption Strategies
4.8 Case Studies Analysis
Chapter 5: Conclusion and Summary
5.1 Summary of Findings
5.2 Conclusion
5.3 Recommendations for Practitioners
5.4 Future Research Directions
Thesis Overview:
Data engineering strategies are crucial for building scalable data lakes to manage and analyze large volumes of data effectively. This thesis explores various challenges and opportunities in data engineering for data lakes, including data ingestion, data quality, data governance, and data processing. By examining best practices, emerging technologies, and case studies of successful implementations, this research aims to provide insights and recommendations for organizations looking to leverage data lakes for data analytics and business intelligence applications. The findings from this study will contribute to advancing the field of data engineering and help organizations optimize their data lake infrastructure for improved data management and analytics capabilities.
[ad_2]
Purchase Detail
Download the complete project materials to this project with Abstract, Chapters 1 – 5, References and Appendix (Questionaire, Charts, etc), Click Here to place an order via whatsapp. Got question or enquiry; Click here to chat us up via Whatsapp.
You can also call 08111770269 or +2348059541956 to place an order or use the whatsapp button below to chat us up.
Bank details are stated below.
Bank: UBA
Account No: 1021412898
Account Name: Starnet Innovations Limited
The Blazingprojects Mobile App
Download and install the Blazingprojects Mobile App from Google Play to enjoy over 50,000 project topics and materials from 73 departments, completely offline (no internet needed) with monthly update to topics, click here to install.