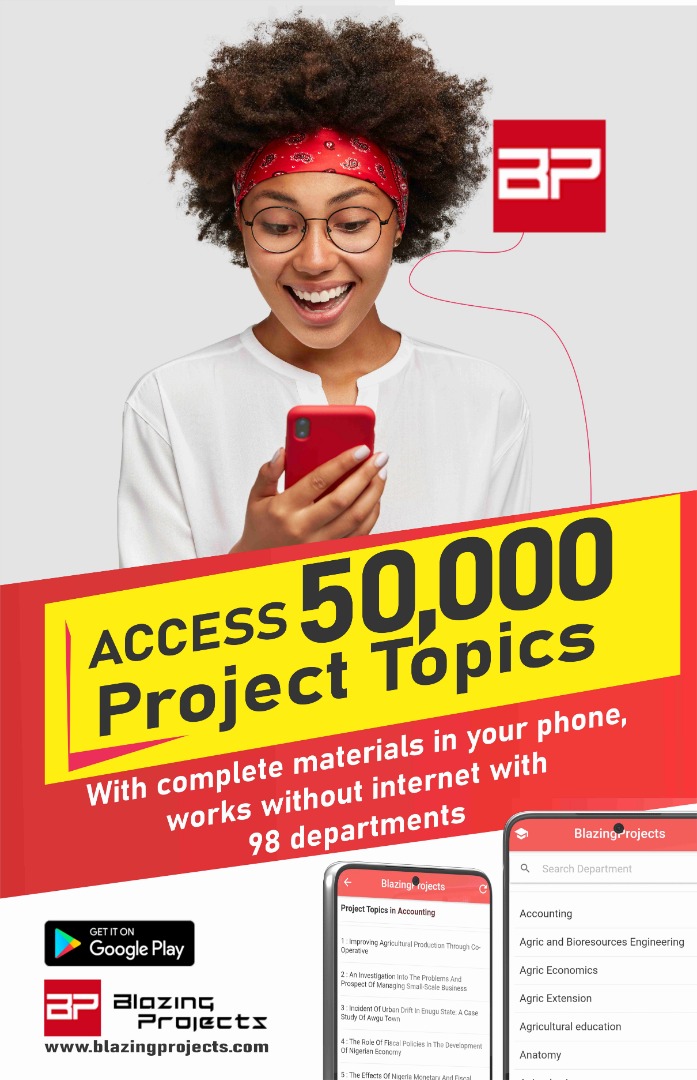
Introduction
In recent years, the field of computational neuroscience has seen tremendous growth with the advancement of technology and the increasing understanding of the complex mechanisms underlying neural circuits and networks. Computational modeling has emerged as a powerful tool in the study of neural systems, allowing researchers to simulate and analyze the behavior of these intricate networks in silico. By incorporating principles from mathematics, physics, and computer science, computational modeling provides a unique perspective on the functioning of the brain and can help unravel the mysteries of neurological disorders and cognitive processes.
This thesis focuses on the computational modeling of neural circuits and networks, aiming to provide insights into the underlying mechanisms governing brain function. By developing mathematical models and simulation algorithms, researchers can gain a better understanding of how individual neurons interact with each other to form complex networks, how information is processed and transmitted within these networks, and how disruptions in these processes can lead to neurological diseases. Through computational modeling, researchers can explore hypotheses, test predictions, and gain new perspectives on the functioning of the brain.
Chapter 1: Introduction
1.1 Introduction
1.2 Background of study
1.3 Problem Statement
1.4 Objective of study
1.5 Limitation of study
1.6 Scope of study
1.7 Significance of study
1.8 Structure of the Thesis
1.9 Definition of terms
Chapter 2: Literature Review
2.1 Overview of computational neuroscience
2.2 Neural circuits and network modeling
2.3 Types of neural network models
2.4 Applications of computational modeling in neuroscience
2.5 Computational tools for neural network simulation
2.6 Challenges in neural network modeling
2.7 Biological basis of neural circuits
2.8 Computational approaches to studying neural dynamics
2.9 Neural plasticity and learning
2.10 Integration of experimental and computational approaches in neuroscience research
Chapter 3: Research Methodology
3.1 Data collection and preprocessing
3.2 Model development and validation
3.3 Simulation techniques
3.4 Parameter estimation methods
3.5 Network connectivity analysis
3.6 Statistical analysis of simulation results
3.7 Sensitivity analysis
3.8 Validation of computational models
3.9 Comparison with experimental data
Chapter 4: Discussion of Findings
4.1 Analysis of simulation results
4.2 Interpretation of model predictions
4.3 Comparison with existing literature
4.4 Implications for neuroscience research
4.5 Limitations of the study
4.6 Future directions
4.7 Recommendations for further research
4.8 Practical applications of computational modeling in neuroscience
Chapter 5: Conclusion and Summary
5.1 Recap of key findings
5.2 Contributions to the field of computational neuroscience
5.3 Implications for understanding neural circuits and networks
5.4 Future prospects for computational modeling in neuroscience
5.5 Conclusion and final remarks
5.6 Recommendations for future studies
Thesis Overview on Computational Modeling of Neural Circuits and Networks
The field of computational neuroscience has made significant strides in recent years, leveraging advances in technology and computational methods to unravel the complexities of neural circuits and networks. By developing mathematical models and simulation algorithms, researchers can simulate the behavior of neural systems and gain insights into the mechanisms governing brain function. This thesis focuses on the computational modeling of neural circuits and networks, aiming to explore the interactions between individual neurons, the processing of information within neural networks, and the implications for neurological disorders.
Chapter 1 provides an introduction to the topic, outlining the background, problem statement, objectives, limitations, scope, significance, and structure of the thesis. Chapter 2 presents a comprehensive literature review, covering the principles of computational neuroscience, neural circuit modeling, types of neural network models, applications of computational modeling in neuroscience, and challenges in neural network modeling. Chapter 3 details the research methodology, including data collection, model development, simulation techniques, parameter estimation, network connectivity analysis, and validation strategies.
In Chapter 4, the discussion of findings analyzes the simulation results, interprets model predictions, compares with existing literature, and explores the implications for neuroscience research. The chapter also addresses the limitations of the study, suggests future directions, recommends further research, and discusses the practical applications of computational modeling in neuroscience. Chapter 5 presents the conclusion and summary, recapping key findings, highlighting contributions to the field, discussing implications for understanding neural circuits and networks, outlining future prospects, and providing recommendations for future studies. Overall, this thesis aims to advance the field of computational neuroscience and contribute to the understanding of neural systems through innovative modeling approaches.
Purchase Detail
Download the complete project materials to this project with Abstract, Chapters 1 – 5, References and Appendix (Questionaire, Charts, etc), Click Here to place an order via whatsapp. Got question or enquiry; Click here to chat us up via Whatsapp.
You can also call 08111770269 or +2348059541956 to place an order or use the whatsapp button below to chat us up.
Bank details are stated below.
Bank: UBA
Account No: 1021412898
Account Name: Starnet Innovations Limited
The Blazingprojects Mobile App
Download and install the Blazingprojects Mobile App from Google Play to enjoy over 50,000 project topics and materials from 73 departments, completely offline (no internet needed) with monthly update to topics, click here to install.