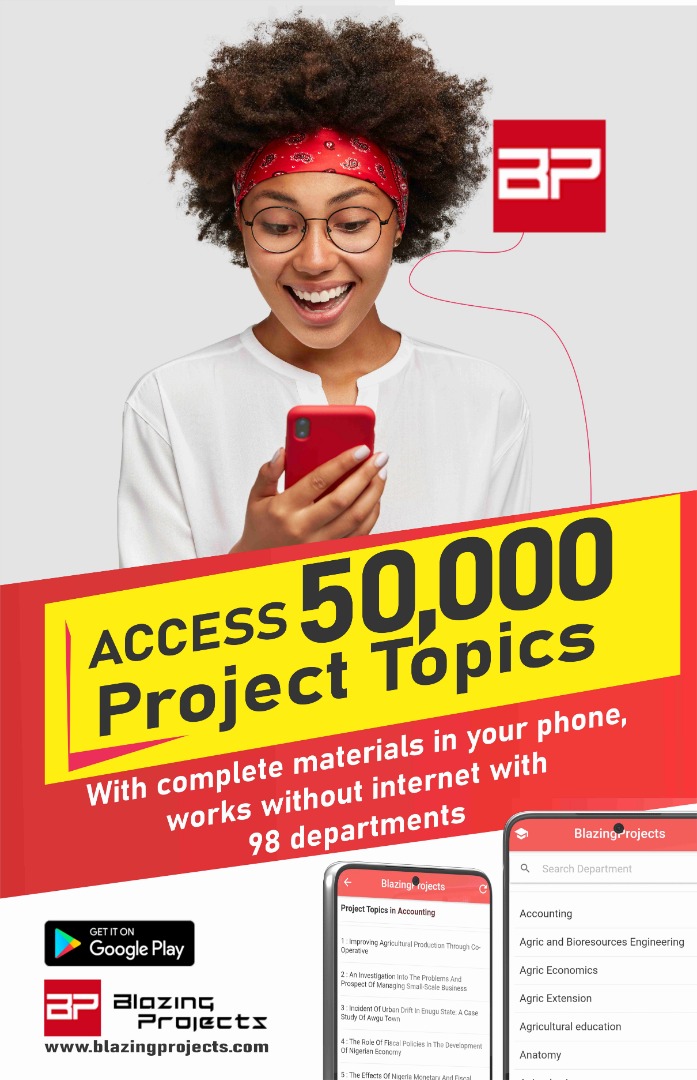
Introduction
Automatic speech recognition (ASR) has become an integral part of our daily lives, from virtual assistants on our smartphones to automated customer service systems. However, most ASR systems are designed with a bias towards standard accents and languages, leading to challenges when recognizing speech with varied accents and languages. This thesis aims to explore and develop ASR models that are adapted for varied accents and languages, to improve the accuracy and performance of speech recognition technology in diverse linguistic contexts.
Chapter 1: Introduction
1.1 Introduction
1.2 Background of Study
1.3 Problem Statement
1.4 Objective of Study
1.5 Limitation of Study
1.6 Scope of Study
1.7 Significance of Study
1.8 Structure of the Thesis
1.9 Definition of Terms
Chapter 2: Literature Review
2.1 Overview of Automatic Speech Recognition
2.2 Challenges in ASR for Varied Accents and Languages
2.3 Existing Approaches to Adapt ASR for Varied Accents and Languages
2.4 Machine Learning Techniques in ASR
2.5 Deep Learning Models for ASR
2.6 Transfer Learning in ASR
2.7 Accent Detection and Language Identification
2.8 Evaluation Metrics for ASR
2.9 Case Studies on ASR Adaptation
2.10 Future Trends in ASR for Varied Accents and Languages
Chapter 3: Research Methodology
3.1 Research Design
3.2 Data Collection and Preprocessing
3.3 Feature Extraction
3.4 Model Development
3.5 Training and Evaluation
3.6 Performance Metrics
3.7 Cross-validation Techniques
3.8 Ethical Considerations
Chapter 4: Discussion of Findings
4.1 Performance Comparison of ASR Models
4.2 Impact of Accent Adaptation on Recognition Accuracy
4.3 Language Adaptation Techniques
4.4 Error Analysis and Improvement Strategies
4.5 Model Generalization Across Accents and Languages
Chapter 5: Conclusion and Summary
5.1 Summary of Findings
5.2 Contributions to the Field
5.3 Implications for Future Research
5.4 Practical Applications of ASR for Varied Accents and Languages
Thesis Overview
Automatic speech recognition (ASR) technology has revolutionized the way we interact with devices and systems by enabling machines to understand and interpret human speech. However, existing ASR systems are often biased towards standard accents and languages, leading to challenges in accurately recognizing speech with varied accents and languages. This thesis aims to address this gap by exploring and developing ASR models that are adapted for varied accents and languages, to enhance the performance and accuracy of speech recognition technology in diverse linguistic contexts.
In Chapter 1, the introduction provides an overview of the research topic, highlighting the background, problem statement, objectives, limitations, scope, significance, structure of the thesis, and definition of key terms. Chapter 2 reviews relevant literature on ASR, challenges in adapting ASR for varied accents and languages, existing approaches, machine learning techniques, deep learning models, transfer learning, accent detection, language identification, evaluation metrics, and future trends in ASR.
Chapter 3 outlines the research methodology, including research design, data collection and preprocessing, feature extraction, model development, training and evaluation, performance metrics, cross-validation techniques, and ethical considerations. Chapter 4 discusses the findings of the study, including performance comparison of ASR models, impact of accent adaptation, language adaptation techniques, error analysis, and model generalization across accents and languages.
Finally, Chapter 5 presents the conclusion and summary of the thesis, highlighting the key findings, contributions to the field, implications for future research, and practical applications of ASR for varied accents and languages. This thesis aims to contribute to the advancement of ASR technology by enhancing its adaptability to diverse linguistic contexts, thereby improving the overall user experience and accessibility of speech recognition technology.
Purchase Detail
Download the complete project materials to this project with Abstract, Chapters 1 – 5, References and Appendix (Questionaire, Charts, etc), Click Here to place an order via whatsapp. Got question or enquiry; Click here to chat us up via Whatsapp.
You can also call 08111770269 or +2348059541956 to place an order or use the whatsapp button below to chat us up.
Bank details are stated below.
Bank: UBA
Account No: 1021412898
Account Name: Starnet Innovations Limited
The Blazingprojects Mobile App
Download and install the Blazingprojects Mobile App from Google Play to enjoy over 50,000 project topics and materials from 73 departments, completely offline (no internet needed) with monthly update to topics, click here to install.