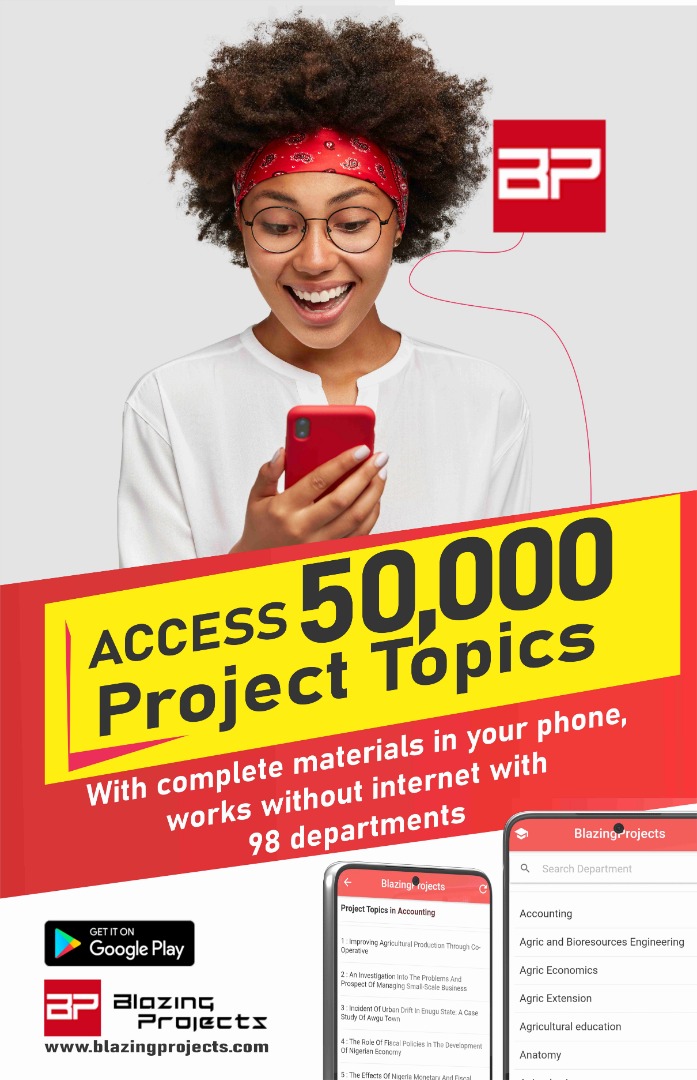
[ad_1]
Introduction:
Anomaly detection in financial transactions plays a crucial role in identifying and preventing fraudulent activities that pose significant risks to financial institutions and their customers. Traditional methods of anomaly detection often fall short in accurately identifying complex patterns of fraud in large-scale datasets. In recent years, graph analysis and unsupervised learning techniques have emerged as powerful tools for detecting anomalies in financial transactions by modeling the relationships between entities and transactions in a network. This thesis aims to investigate the use of graph analysis and unsupervised learning algorithms for anomaly detection in financial transactions.
1.1 Introduction
1.2 Background of Study
1.3 Problem Statement
1.4 Objectives of Study
1.5 Limitations of Study
1.6 Scope of Study
1.7 Significance of Study
1.8 Structure of the Thesis
1.9 Definition of Terms
Chapter Two: Literature Review
2.1 Overview of Anomaly Detection in Financial Transactions
2.2 Traditional Methods of Anomaly Detection
2.3 Graph Analysis Techniques
2.4 Unsupervised Learning Algorithms
2.5 Applications of Graph Analysis in Finance
2.6 Challenges in Anomaly Detection in Financial Transactions
2.7 Recent Advances in Anomaly Detection Techniques
2.8 Comparative Analysis of Anomaly Detection Techniques
2.9 Theoretical Frameworks in Anomaly Detection
2.10 Summary of Literature Review
Chapter Three: Research Methodology
3.1 Research Design
3.2 Data Collection and Preprocessing
3.3 Graph Construction
3.4 Feature Engineering
3.5 Unsupervised Learning Algorithms
3.6 Model Evaluation Metrics
3.7 Experiment Design
3.8 Software Tools and Technologies
3.9 Ethical Considerations in Data Analysis
Chapter Four: Discussion of Findings
4.1 Performance Evaluation of Anomaly Detection Models
4.2 Detection of Fraudulent Patterns in Financial Transactions
4.3 Interpretation of Graph Analysis Results
4.4 Comparative Analysis of Unsupervised Learning Algorithms
4.5 Real-World Applications of Anomaly Detection Models
4.6 Implications for Financial Institutions
4.7 Limitations of Anomaly Detection Techniques
4.8 Recommendations for Future Research
Chapter Five: Conclusion and Summary
5.1 Summary of Findings
5.2 Contributions of the Study
5.3 Practical Implications
5.4 Theoretical Implications
5.5 Conclusion
5.6 Future Research Directions
Thesis Overview:
The detection of anomalies in financial transactions is a critical task for financial institutions to ensure the security and integrity of their operations. Traditional methods of anomaly detection often rely on predefined rules or thresholds, which may not be effective in identifying complex fraudulent activities. In this thesis, we explore the use of graph analysis and unsupervised learning techniques to detect anomalies in financial transactions.
The research begins with a comprehensive literature review on anomaly detection in financial transactions, highlighting the limitations of traditional methods and the potential of graph analysis and unsupervised learning algorithms. The methodology chapter details the research design, data collection, preprocessing, graph construction, feature engineering, and model evaluation metrics used in the study.
The discussion of findings chapter presents the performance evaluation of anomaly detection models, the detection of fraudulent patterns in financial transactions, interpretation of graph analysis results, and real-world applications of the models. The conclusion and summary chapter summarizes the findings, contributions of the study, practical and theoretical implications, and recommendations for future research.
Overall, this thesis contributes to the growing body of research on anomaly detection in financial transactions by demonstrating the effectiveness of graph analysis and unsupervised learning techniques in identifying fraudulent activities. The findings of this study have important implications for financial institutions in improving their fraud detection systems and enhancing the security of financial transactions.
[ad_2]
Purchase Detail
Download the complete project materials to this project with Abstract, Chapters 1 – 5, References and Appendix (Questionaire, Charts, etc), Click Here to place an order via whatsapp. Got question or enquiry; Click here to chat us up via Whatsapp.
You can also call 08111770269 or +2348059541956 to place an order or use the whatsapp button below to chat us up.
Bank details are stated below.
Bank: UBA
Account No: 1021412898
Account Name: Starnet Innovations Limited
The Blazingprojects Mobile App
Download and install the Blazingprojects Mobile App from Google Play to enjoy over 50,000 project topics and materials from 73 departments, completely offline (no internet needed) with monthly update to topics, click here to install.