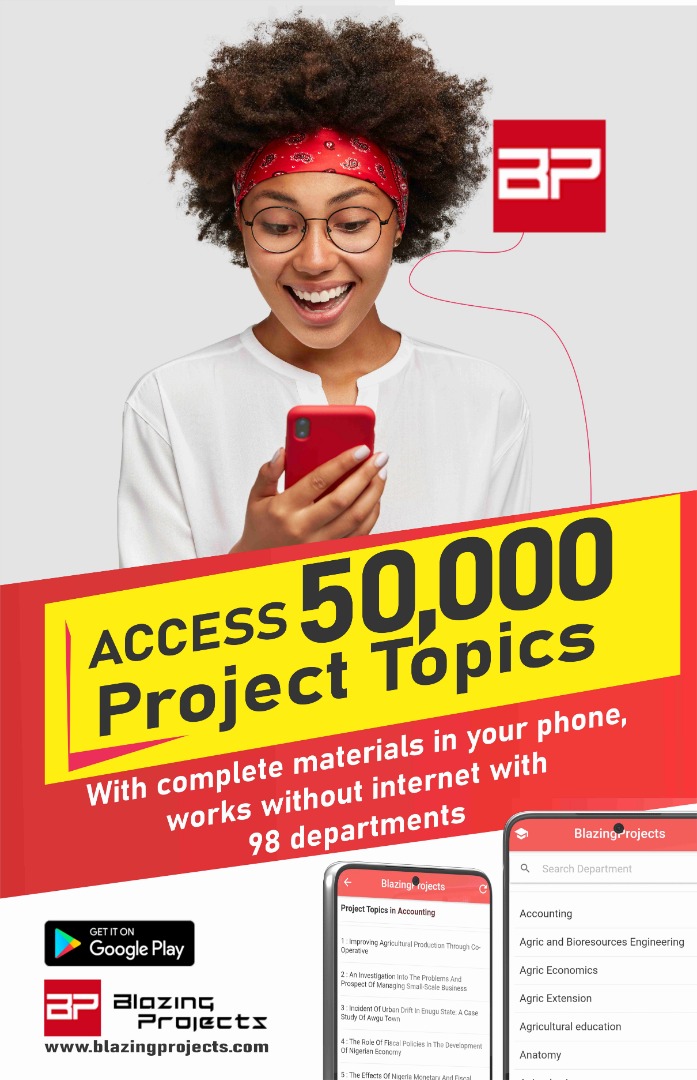
[ad_1]
Introduction
Customer churn prediction in the retail banking industry is a crucial area of research due to the high competition among banks, the increasing customer expectations, and the ease of switching between financial institutions. Identifying customers who are at risk of churning allows banks to implement targeted retention strategies, ultimately leading to increased customer loyalty and profitability. In recent years, the availability of transaction data and advancements in machine learning techniques have provided new opportunities for accurate and efficient churn prediction in the banking sector.
1.1 Introduction
1.2 Background of the Study
1.3 Problem Statement
1.4 Objective of the Study
1.5 Limitation of the Study
1.6 Scope of the Study
1.7 Significance of the Study
1.8 Structure of the Thesis
1.9 Definition of Terms
Chapter 2: Literature Review
2.1 Customer Churn in the Retail Banking Industry
2.2 Predictive Analytics in Banking
2.3 Transaction Data Analysis
2.4 Machine Learning Techniques
2.5 Previous Studies on Customer Churn Prediction
2.6 Factors Influencing Customer Churn
2.7 Retention Strategies in Banking
2.8 Evaluation Metrics for Churn Prediction Models
2.9 Challenges in Churn Prediction
2.10 Future Trends in Customer Churn Prediction
Chapter 3: Research Methodology
3.1 Data Collection
3.2 Data Preprocessing
3.3 Feature Selection
3.4 Model Selection
3.5 Model Training
3.6 Model Evaluation
3.7 Hyperparameter Tuning
3.8 Cross-validation
3.9 Performance Metrics
Chapter 4: Discussion of Findings
4.1 Descriptive Analysis of the Data
4.2 Feature Importance
4.3 Model Performance Comparison
4.4 Interpretation of Results
4.5 Insights for Churn Prevention
4.6 Recommendations for Banks
4.7 Future Research Directions
Chapter 5: Conclusion and Summary
5.1 Summary of Findings
5.2 Contributions of the Study
5.3 Implications for Practice
5.4 Limitations of the Study
5.5 Recommendations for Future Research
5.6 Conclusion
Thesis Overview
The retail banking industry is undergoing significant transformation due to technological advancements and changing customer preferences. One of the critical challenges facing banks today is customer churn, where customers switch their accounts to competitors, leading to revenue loss and decreased customer loyalty. In this thesis, we focus on customer churn prediction in the retail banking industry using transaction data and machine learning techniques.
The introduction provides an overview of the importance of customer churn prediction in banking and the research objectives. The literature review discusses previous studies on customer churn, predictive analytics in banking, and machine learning techniques for churn prediction. The research methodology describes the data collection, preprocessing, model selection, and evaluation processes.
The discussion of findings presents the results of the churn prediction model, including feature importance, model performance, and recommendations for banks. The conclusion summarizes the key findings, contributions of the study, implications for practice, and recommendations for future research. Overall, this thesis aims to provide valuable insights for banks to improve customer retention and profitability in the highly competitive retail banking industry.
[ad_2]
Purchase Detail
Download the complete project materials to this project with Abstract, Chapters 1 – 5, References and Appendix (Questionaire, Charts, etc), Click Here to place an order via whatsapp. Got question or enquiry; Click here to chat us up via Whatsapp.
You can also call 08111770269 or +2348059541956 to place an order or use the whatsapp button below to chat us up.
Bank details are stated below.
Bank: UBA
Account No: 1021412898
Account Name: Starnet Innovations Limited
The Blazingprojects Mobile App
Download and install the Blazingprojects Mobile App from Google Play to enjoy over 50,000 project topics and materials from 73 departments, completely offline (no internet needed) with monthly update to topics, click here to install.