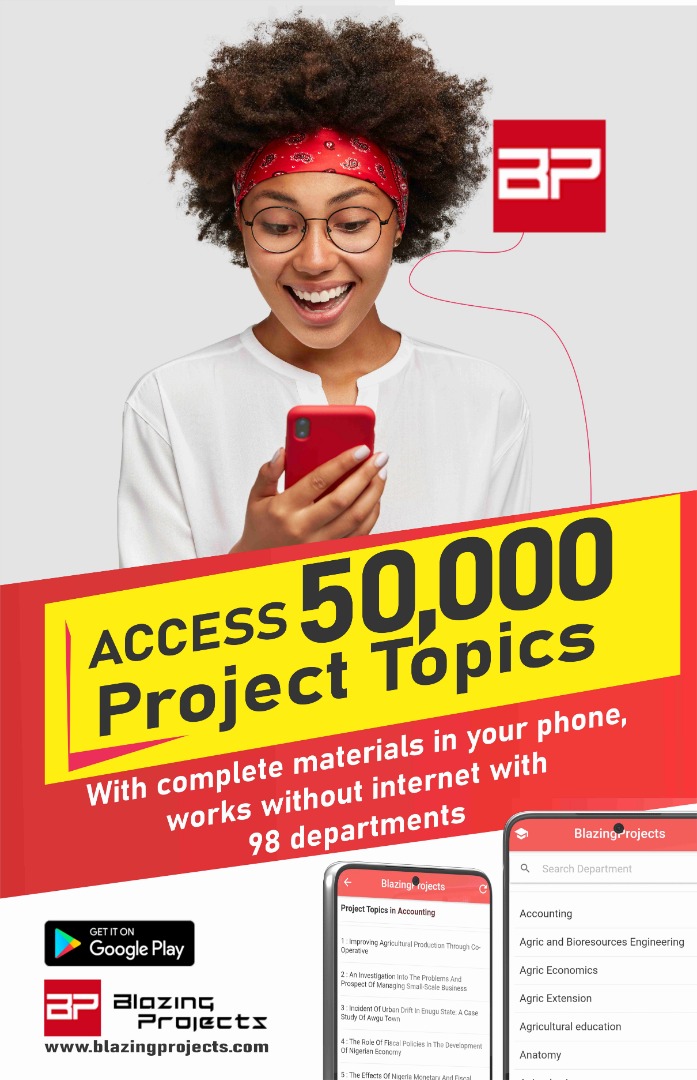
[ad_1]
Introduction:
Traffic congestion poses a significant challenge to logistics companies, leading to delays, increased costs, and decreased customer satisfaction. Traditional methods of predicting and managing congestion have shown limitations in providing accurate and real-time information. With the advancements in deep learning and the availability of fleet GPS data, there is an opportunity to develop more efficient and accurate prediction models for traffic congestion.
This thesis aims to explore the use of deep learning techniques and fleet GPS data for predicting traffic congestion to optimize logistics operations. By developing a predictive model that can anticipate congestion patterns, logistics companies can better plan their routes, reduce delivery times, and improve overall operational efficiency.
Chapter 1: Introduction
1.1 Introduction
1.2 Background of study
1.3 Problem Statement
1.4 Objective of study
1.5 Limitation of study
1.6 Scope of study
1.7 Significance of study
1.8 Structure of the Thesis
1.9 Definition of terms
Chapter 2: Literature Review
2.1 Introduction to traffic congestion
2.2 Traditional methods for predicting traffic congestion
2.3 Deep learning techniques for traffic prediction
2.4 Use of fleet GPS data in logistics optimization
2.5 Integration of deep learning and GPS data for congestion prediction
2.6 Related studies on traffic congestion prediction
2.7 Challenges in traffic congestion prediction
2.8 Opportunities for improvement in logistics optimization
2.9 Summary of literature review
2.10 Conceptual framework
Chapter 3: Research Methodology
3.1 Introduction
3.2 Research design
3.3 Data collection methods
3.4 Data preprocessing techniques
3.5 Deep learning model selection
3.6 Feature selection and engineering
3.7 Model training and evaluation
3.8 Performance metrics
3.9 Ethical considerations
3.10 Summary of research methodology
Chapter 4: Discussion of Findings
4.1 Introduction
4.2 Data analysis results
4.3 Model performance evaluation
4.4 Comparison with existing methods
4.5 Insights into congestion patterns
4.6 Implications for logistics optimization
4.7 Limitations of the study
4.8 Future research directions
4.9 Recommendations for practical implementation
4.10 Summary of findings
Chapter 5: Conclusion and Summary
5.1 Summary of the study
5.2 Achievements of the research
5.3 Contributions to the field
5.4 Implications for logistics industry
5.5 Limitations of the study
5.6 Suggestions for future research
5.7 Conclusion
5.8 Recommendations for further study
Thesis Overview:
Traffic congestion is a prevalent issue in urban areas, leading to delays in logistics operations. This thesis focuses on the prediction of traffic congestion using deep learning techniques and fleet GPS data to optimize logistics operations. The introduction provides background information on the problem statement, objectives, limitations, scope, significance, and structure of the thesis.
The literature review delves into traditional methods for predicting traffic congestion, deep learning techniques, fleet GPS data utilization in logistics optimization, and related studies. It also discusses challenges, opportunities, and establishes a conceptual framework.
The research methodology chapter describes the research design, data collection, preprocessing, model selection, feature engineering, training, evaluation, performance metrics, ethical considerations, and summarizes the methodology.
The discussion of findings chapter presents data analysis results, model performance evaluation, comparison with existing methods, insights into congestion patterns, implications for logistics optimization, limitations, future research directions, and recommendations for practical implementation.
Finally, the conclusion and summary chapter provide a comprehensive overview of the study, achievements, contributions, implications, limitations, suggestions for future research, and recommendations for further study. Through this thesis, we aim to provide valuable insights for improving logistics operations through traffic congestion prediction using deep learning and fleet GPS data.
[ad_2]
Purchase Detail
Download the complete project materials to this project with Abstract, Chapters 1 – 5, References and Appendix (Questionaire, Charts, etc), Click Here to place an order via whatsapp. Got question or enquiry; Click here to chat us up via Whatsapp.
You can also call 08111770269 or +2348059541956 to place an order or use the whatsapp button below to chat us up.
Bank details are stated below.
Bank: UBA
Account No: 1021412898
Account Name: Starnet Innovations Limited
The Blazingprojects Mobile App
Download and install the Blazingprojects Mobile App from Google Play to enjoy over 50,000 project topics and materials from 73 departments, completely offline (no internet needed) with monthly update to topics, click here to install.