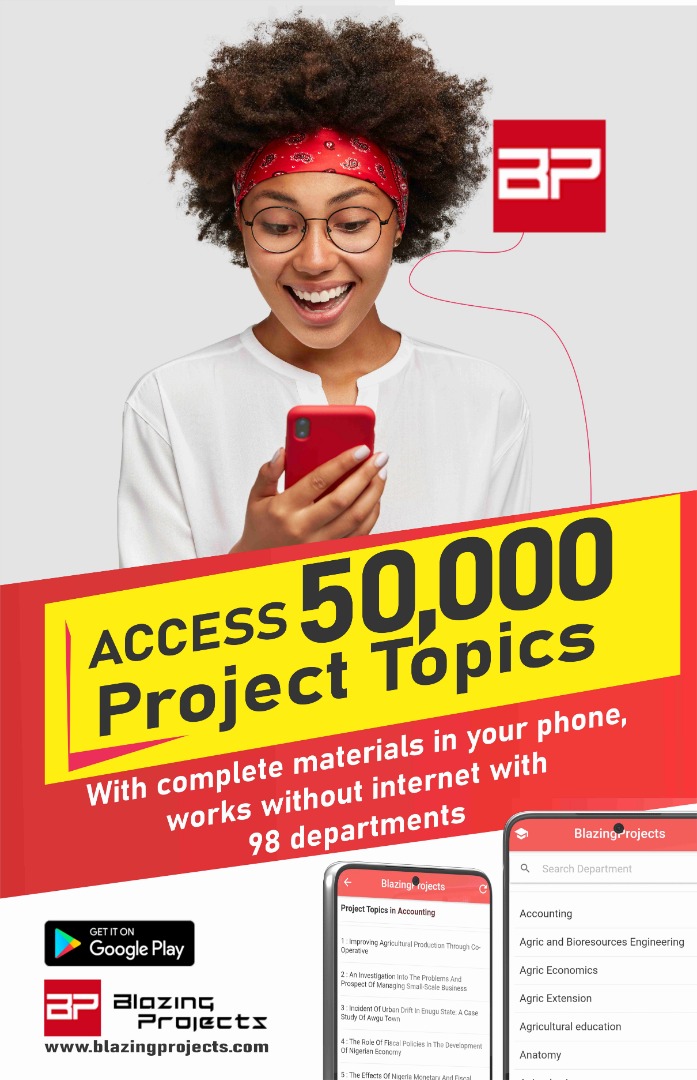
[ad_1]
Introduction:
Anomaly detection plays a critical role in ensuring the security and reliability of industrial control systems (ICS), particularly in the context of Supervisory Control and Data Acquisition (SCADA) systems. SCADA systems are widely used in industries such as power plants, water treatment facilities, and manufacturing plants to monitor and control the processes. However, these systems are vulnerable to cyber-attacks and malfunctions, which can lead to catastrophic consequences. Therefore, the ability to detect anomalies in SCADA data in real-time is essential for ensuring the safe and efficient operation of these critical infrastructure systems.
This thesis focuses on the use of unsupervised learning techniques for anomaly detection in SCADA data. Unsupervised learning is particularly well-suited for this task as it does not require labeled data for training, making it suitable for detecting unknown and novel anomalies. By leveraging the inherent patterns and structures within the SCADA data, unsupervised learning algorithms can identify deviations from normal behavior that may indicate a potential security breach or equipment malfunction.
Chapter 1: Introduction
1.1 Introduction
1.2 Background of study
1.3 Problem Statement
1.4 Objective of study
1.5 Limitation of study
1.6 Scope of study
1.7 Significance of study
1.8 Structure of the Thesis
1.9 Definition of Terms
Chapter 2: Literature Review
2.1 Overview of Anomaly Detection in ICS
2.2 SCADA Systems and Their Vulnerabilities
2.3 Unsupervised Learning Techniques for Anomaly Detection
2.4 Existing Approaches to Anomaly Detection in SCADA Data
2.5 Case Studies on Anomaly Detection in ICS
2.6 Challenges and Limitations of Current Methods
2.7 Recent Advances in Anomaly Detection Technologies
2.8 Importance of Real-time Anomaly Detection in ICS
2.9 The Role of Data Preprocessing in Anomaly Detection
2.10 Future Directions in Anomaly Detection Research
Chapter 3: Research Methodology
3.1 Data Collection and Preprocessing
3.2 Selection of Unsupervised Learning Algorithms
3.3 Evaluation Metrics for Anomaly Detection
3.4 Experimental Setup
3.5 Performance Benchmarks
3.6 Model Interpretability and Explainability
3.7 Parameter Tuning and Optimization
3.8 Cross-validation Techniques
Chapter 4: Discussion of Findings
4.1 Analysis of Anomaly Detection Results
4.2 Comparison of Different Unsupervised Learning Algorithms
4.3 Interpretation of Detected Anomalies
4.4 Impact of Data Preprocessing Techniques
4.5 Robustness and Scalability of the Anomaly Detection Models
4.6 Practical Considerations for Implementation
4.7 Limitations and Future Research Directions
4.8 Case Studies and Use Cases
4.9 Recommendations for Industry Practitioners
4.10 Ethical and Security Implications
Chapter 5: Conclusion and Summary
5.1 Summary of Key Findings
5.2 Contribution to the Field
5.3 Implications for Industrial Applications
5.4 Future Research Directions
5.5 Conclusion
Thesis Overview:
Anomaly detection in industrial control systems (ICS) using SCADA data and unsupervised learning is a critical area of research that aims to enhance the security and reliability of critical infrastructure systems. This thesis focuses on the development and evaluation of unsupervised learning algorithms for detecting anomalies in SCADA data, with the ultimate goal of improving the resilience of ICS against cyber-attacks and malfunctions.
Chapter 1 provides an introduction to the research topic, outlining the background, problem statement, objectives, scope, significance, and structure of the thesis. Chapter 2 presents a comprehensive literature review on anomaly detection in ICS, SCADA systems, unsupervised learning techniques, existing approaches, challenges, recent advances, and future directions. Chapter 3 details the research methodology, including data collection and preprocessing, algorithm selection, evaluation metrics, experimental setup, performance benchmarks, and model interpretability.
Chapter 4 offers a detailed discussion of the findings, including an analysis of anomaly detection results, comparisons of different algorithms, interpretation of anomalies, impact of preprocessing, robustness, scalability, implications for practitioners, limitations, case studies, and recommendations. Chapter 5 concludes the thesis by summarizing key findings, contributions, implications, future research directions, and a final conclusion on the importance of anomaly detection in ICS for enhancing system security and reliability.
[ad_2]
Purchase Detail
Download the complete project materials to this project with Abstract, Chapters 1 – 5, References and Appendix (Questionaire, Charts, etc), Click Here to place an order via whatsapp. Got question or enquiry; Click here to chat us up via Whatsapp.
You can also call 08111770269 or +2348059541956 to place an order or use the whatsapp button below to chat us up.
Bank details are stated below.
Bank: UBA
Account No: 1021412898
Account Name: Starnet Innovations Limited
The Blazingprojects Mobile App
Download and install the Blazingprojects Mobile App from Google Play to enjoy over 50,000 project topics and materials from 73 departments, completely offline (no internet needed) with monthly update to topics, click here to install.