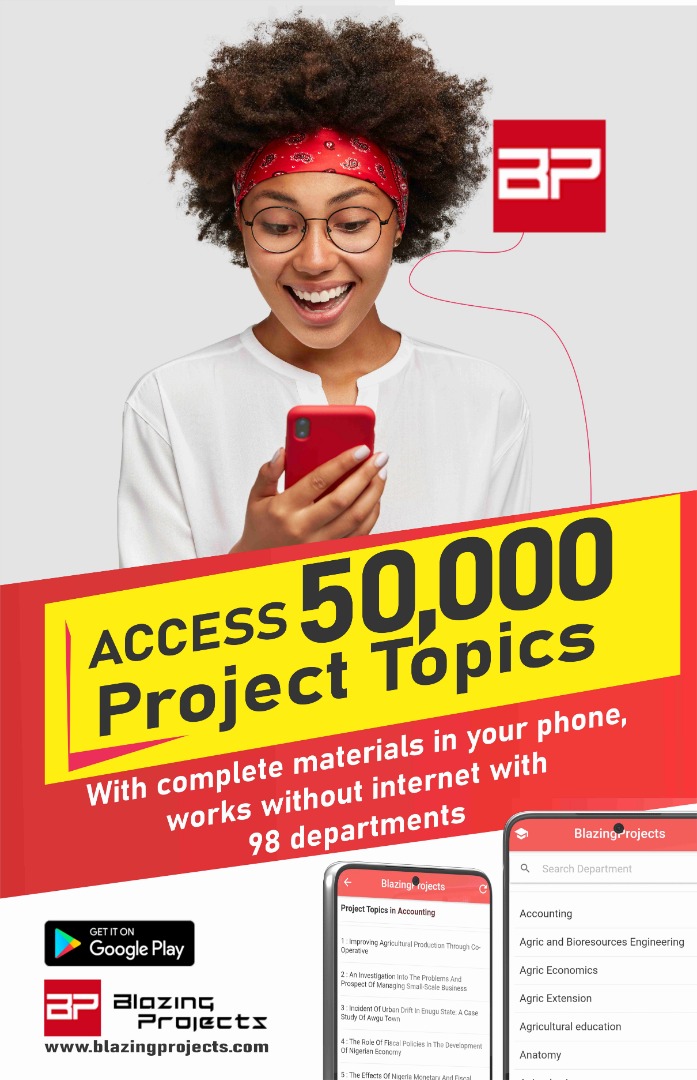
[ad_1]
Introduction:
With the increasing popularity of streaming services in the entertainment industry, customer retention has become a critical issue for streaming video providers. Customer churn, or the rate at which subscribers cancel their subscriptions, is a significant concern as it directly impacts revenue and profitability. To address this challenge, many streaming video platforms are turning to data analytics and machine learning techniques to predict customer churn and take proactive measures to retain subscribers. In particular, viewer engagement data such as watching habits, viewing frequency, and content preferences have proven to be valuable predictors of churn behavior.
This thesis focuses on customer churn prediction in the streaming video industry using viewer engagement data and machine learning algorithms. By leveraging advanced analytical techniques, this study aims to develop a model that can accurately forecast the likelihood of a viewer cancelling their subscription. The insights gained from this research can help streaming video providers to tailor their content offerings, marketing strategies, and customer service initiatives to reduce churn rates and improve customer retention.
Table of Contents:
1. Introduction
1.1 Introduction
1.2 Background of the study
1.3 Problem Statement
1.4 Objective of the study
1.5 Limitation of the study
1.6 Scope of the study
1.7 Significance of the study
1.8 Structure of the Thesis
1.9 Definition of Terms
2. Literature Review
2.1 Overview of Customer Churn Prediction
2.2 Streaming Video Industry Trends
2.3 Viewer Engagement Data
2.4 Machine Learning for Churn Prediction
2.5 Previous Studies on Customer Churn in Streaming Services
2.6 Factors Influencing Customer Churn
2.7 Strategies for Customer Retention
2.8 Data Analytics in the Entertainment Industry
2.9 Challenges in Churn Prediction
2.10 Summary of Literature Review
3. Research Methodology
3.1 Research Design
3.2 Data Collection
3.3 Variable Selection
3.4 Data Preprocessing
3.5 Machine Learning Algorithms
3.6 Model Evaluation
3.7 Cross-validation
3.8 Hypothesis Testing
3.9 Ethical Considerations
4. Discussion of Findings
4.1 Descriptive Statistics
4.2 Churn Prediction Model Performance
4.3 Feature Importance Analysis
4.4 Comparison with Existing Models
4.5 Implications for Streaming Video Providers
4.6 Managerial Recommendations
4.7 Future Research Directions
5. Conclusion and Summary
5.1 Summary of Key Findings
5.2 Contributions to the Literature
5.3 Practical Implications
5.4 Limitations of the Study
5.5 Conclusion
5.6 Recommendations for Future Research
Thesis Overview:
The rapid growth of the streaming video industry has led to fierce competition among providers, with customer retention being a top priority. Customer churn prediction using viewer engagement data and machine learning has emerged as a promising approach to address this challenge. This thesis aims to explore the effectiveness of predictive modeling techniques in forecasting customer churn in the streaming video industry.
Chapter 1 provides an introduction to the research topic, outlining the background, problem statement, objectives, limitations, scope, significance, and structure of the thesis. The chapter also defines key terms related to customer churn prediction and machine learning.
Chapter 2 presents a comprehensive literature review on customer churn prediction, the streaming video industry, viewer engagement data, machine learning algorithms, and previous studies on churn behavior in entertainment services. This section sets the foundation for the research by synthesizing relevant theoretical and empirical knowledge.
Chapter 3 details the research methodology, including the research design, data collection process, variable selection, data preprocessing techniques, machine learning algorithms employed, model evaluation methods, cross-validation procedures, and ethical considerations.
Chapter 4 discusses the findings of the study, including descriptive statistics, churn prediction model performance, feature importance analysis, comparisons with existing models, implications for streaming video providers, managerial recommendations, and suggestions for future research.
Chapter 5 offers a conclusion and summary of the project, highlighting key findings, contributions to the literature, practical implications for industry practitioners, study limitations, and recommendations for future research directions. Overall, this thesis aims to provide valuable insights into the application of predictive analytics in reducing customer churn and enhancing customer retention in the streaming video industry.
[ad_2]
Purchase Detail
Download the complete project materials to this project with Abstract, Chapters 1 – 5, References and Appendix (Questionaire, Charts, etc), Click Here to place an order via whatsapp. Got question or enquiry; Click here to chat us up via Whatsapp.
You can also call 08111770269 or +2348059541956 to place an order or use the whatsapp button below to chat us up.
Bank details are stated below.
Bank: UBA
Account No: 1021412898
Account Name: Starnet Innovations Limited
The Blazingprojects Mobile App
Download and install the Blazingprojects Mobile App from Google Play to enjoy over 50,000 project topics and materials from 73 departments, completely offline (no internet needed) with monthly update to topics, click here to install.