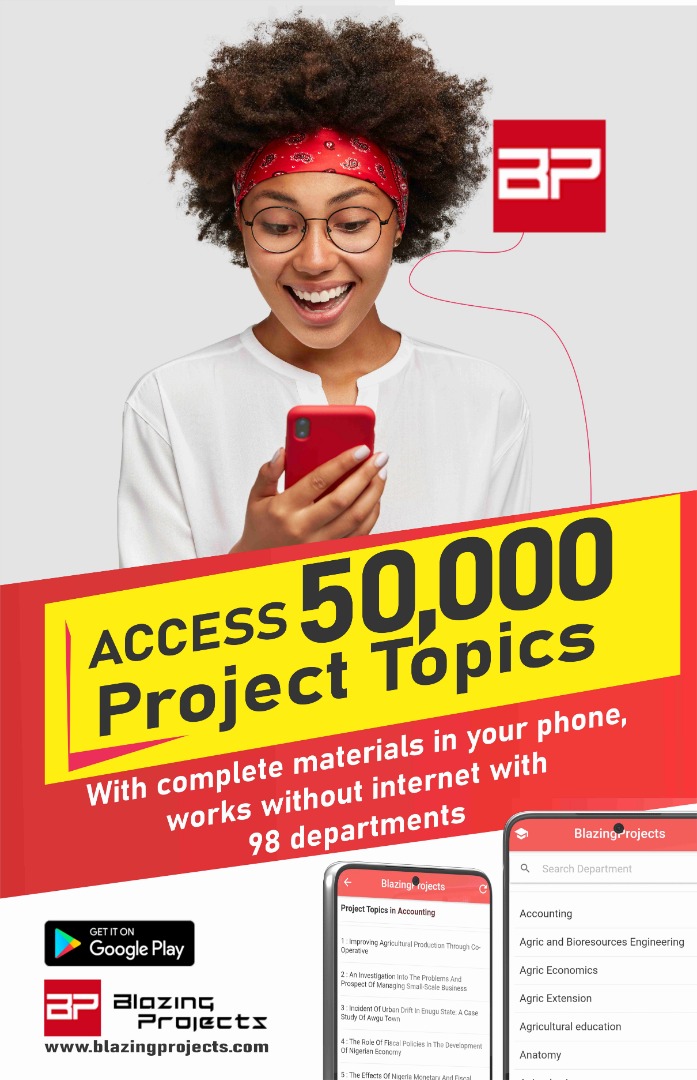
[ad_1]
Introduction
In recent years, wildlife conservation has become an increasingly important issue due to the alarming decline in biodiversity and the threat of extinction facing many species. One of the key challenges in wildlife conservation is the monitoring and tracking of wildlife populations, which is essential for understanding population dynamics, detecting changes in habitat use, and identifying potential threats to species survival. Traditional methods of wildlife monitoring, such as direct observation and radio telemetry, can be time-consuming, labor-intensive, and expensive. In contrast, camera traps offer a cost-effective and efficient way to monitor wildlife populations over large geographic areas.
Camera traps are remote cameras that are triggered by motion or heat sensors to capture images and videos of wildlife in their natural habitats. These devices have revolutionized the field of wildlife monitoring by providing researchers with a wealth of data on animal behavior and population dynamics. However, the sheer volume of data collected by camera traps presents a challenge for efficient analysis and interpretation. Manual analysis of camera trap data is time-consuming and subject to human error, making it impractical for large-scale monitoring projects.
Deep learning, a branch of artificial intelligence that mimics the way the human brain processes information, has shown great promise for automating the analysis of images and videos. By training deep neural networks on labeled datasets of wildlife images, researchers can develop algorithms that are capable of accurately identifying and classifying species in camera trap data. This automated image classification process can significantly reduce the time and effort required to analyze camera trap data, enabling researchers to quickly assess wildlife populations and make informed conservation decisions.
This thesis aims to explore the potential of deep learning for image classification in wildlife conservation using camera trap data. The research will investigate the effectiveness of deep learning algorithms in automatically identifying and classifying wildlife species from camera trap images. The study will also evaluate the strengths and limitations of deep learning in comparison to traditional methods of image analysis. By developing and testing deep learning models on real-world camera trap data, this research seeks to contribute to the advancement of technology for wildlife conservation and provide valuable insights for conservation practitioners.
Chapter 1: Introduction
1.1 Introduction
1.2 Background of study
1.3 Problem Statement
1.4 Objective of study
1.5 Limitation of study
1.6 Scope of study
1.7 Significance of study
1.8 Structure of the Thesis
1.9 Definition of terms
Chapter 2: Literature Review
2.1 Overview of wildlife conservation
2.2 Camera traps in wildlife monitoring
2.3 Deep learning for image classification
2.4 Applications of deep learning in wildlife conservation
2.5 Challenges and limitations of deep learning
2.6 Traditional methods of image analysis
2.7 Previous studies on image classification for wildlife conservation
2.8 Advances in camera trap technology
2.9 Conservation implications of automated image analysis
2.10 Future directions for research
Chapter 3: Research Methodology
3.1 Data collection and preparation
3.2 Deep learning models for image classification
3.3 Training and testing procedures
3.4 Evaluation metrics
3.5 Comparison with traditional methods
3.6 Data analysis techniques
3.7 Ethical considerations
3.8 Software and hardware requirements
Chapter 4: Discussion of Findings
4.1 Performance of deep learning models
4.2 Accuracy and precision of species classification
4.3 Comparison with manual analysis
4.4 Interpretation of results
4.5 Implications for wildlife conservation
4.6 Recommendations for future research
4.7 Practical applications of deep learning
4.8 Limitations and challenges faced
4.9 Contributions to the field of wildlife conservation
Chapter 5: Conclusion and Summary
5.1 Summary of key findings
5.2 Conclusions drawn from the study
5.3 Contributions to knowledge
5.4 Implications for practice
5.5 Recommendations for future research
5.6 Overall significance of the research
Thesis Overview
The rapid advancement of technology in recent years has opened up new possibilities for wildlife conservation, particularly in the realm of data analysis and image classification. This thesis focuses on the application of deep learning techniques for automatically identifying and classifying wildlife species in camera trap data. By harnessing the power of deep neural networks, researchers can streamline the process of analyzing large volumes of camera trap images, enabling more efficient and accurate wildlife monitoring.
The literature review will provide an overview of the current state of wildlife conservation, the use of camera traps in monitoring wildlife populations, and the potential of deep learning for image classification. Previous studies on image analysis for wildlife conservation will be examined to identify gaps in the existing research and highlight the need for further investigation in this area.
The research methodology will outline the data collection and preparation process, the selection of deep learning models for image classification, and the evaluation metrics used to assess the performance of the algorithms. The study will compare the results obtained from deep learning models with those of traditional methods of image analysis to determine the effectiveness and efficiency of deep learning in wildlife conservation.
The discussion of findings will present the results of the study, including the accuracy and precision of species classification, the implications for wildlife conservation, and the practical applications of deep learning in camera trap data analysis. The limitations and challenges faced during the research will be addressed, along with recommendations for future research and the overall contributions to the field of wildlife conservation.
In conclusion, this thesis seeks to demonstrate the potential of deep learning for image classification in wildlife conservation using camera trap data. By developing and testing deep learning models on real-world datasets, this research aims to advance the field of technology for wildlife monitoring and provide valuable insights for conservation practitioners.
[ad_2]
Purchase Detail
Download the complete project materials to this project with Abstract, Chapters 1 – 5, References and Appendix (Questionaire, Charts, etc), Click Here to place an order via whatsapp. Got question or enquiry; Click here to chat us up via Whatsapp.
You can also call 08111770269 or +2348059541956 to place an order or use the whatsapp button below to chat us up.
Bank details are stated below.
Bank: UBA
Account No: 1021412898
Account Name: Starnet Innovations Limited
The Blazingprojects Mobile App
Download and install the Blazingprojects Mobile App from Google Play to enjoy over 50,000 project topics and materials from 73 departments, completely offline (no internet needed) with monthly update to topics, click here to install.