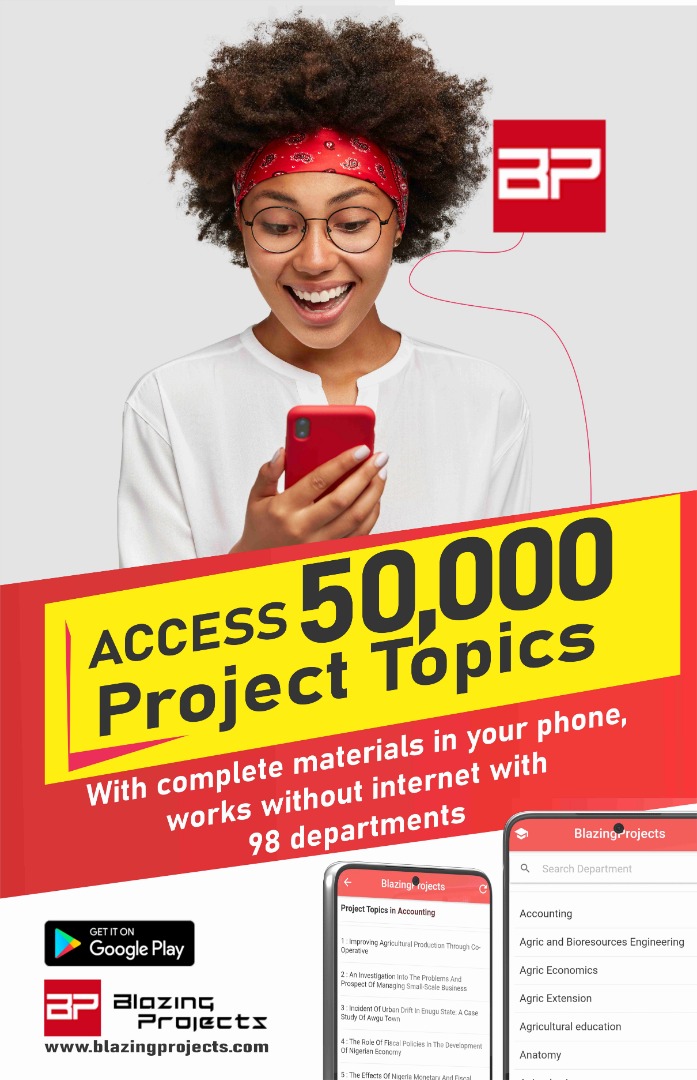
[ad_1]
Introduction
Machine Learning (ML) is a subset of artificial intelligence that aims to develop algorithms that allow computers to learn from and make predictions or decisions based on data. In recent years, ML has gained significant attention in the field of predictive maintenance, particularly in industries like railways where the timely detection of faults and failures is crucial for ensuring the safety and reliability of operations.
Railways are a critical mode of transportation, carrying millions of passengers and tons of freight every day. Ensuring the availability and reliability of railway assets is crucial for maintaining operational efficiency and preventing costly disruptions. Traditional maintenance approaches, such as preventive and reactive maintenance, are often not cost-effective and can lead to unnecessary downtimes and delays. Predictive maintenance, enabled by ML techniques, offers a proactive and data-driven alternative that can help railway operators identify potential issues before they escalate into costly failures.
This thesis aims to explore the application of ML for predictive maintenance in railways. By developing predictive models based on historical and real-time data, railway operators can optimize maintenance schedules, reduce downtime, and improve safety. The following chapters will provide a comprehensive overview of the research conducted in this thesis.
Table of Contents
Chapter 1: Introduction
1.1 Introduction
1.2 Background of study
1.3 Problem Statement
1.4 Objective of study
1.5 Limitation of study
1.6 Scope of study
1.7 Significance of study
1.8 Structure of the Thesis
1.9 Definition of Terms
Chapter 2: Literature Review
2.1 Overview of Predictive Maintenance in Railways
2.2 Applications of Machine Learning in Predictive Maintenance
2.3 Statistical Analysis Techniques
2.4 Sensor Technologies for Condition Monitoring
2.5 Case Studies in Predictive Maintenance
2.6 Challenges and Opportunities
2.7 Integration with Internet of Things (IoT)
2.8 Big Data Analytics in Railway Maintenance
2.9 Predictive Maintenance Algorithms
2.10 Performance Metrics for Predictive Maintenance Models
Chapter 3: Research Methodology
3.1 Research Design
3.2 Data Collection
3.3 Data Preprocessing
3.4 Feature Selection
3.5 Model Selection
3.6 Model Training
3.7 Model Evaluation
3.8 Validation and Testing
3.9 Ethical Considerations
Chapter 4: Discussion of Findings
4.1 Predictive Maintenance Models
4.2 Integration of ML with Railway Maintenance Systems
4.3 Performance Evaluation
4.4 Comparison with Traditional Maintenance Approaches
4.5 Case Studies
4.6 Implementation Challenges
4.7 Cost-Benefit Analysis
4.8 Future Research Directions
Chapter 5: Conclusion and Summary
5.1 Summary of Findings
5.2 Contributions to the Field
5.3 Implications for Practice
5.4 Recommendations for Future Work
5.5 Conclusion
Thesis Overview
Machine Learning for Predictive Maintenance in Railways is a critical research area that aims to leverage advanced data analytics and predictive modeling techniques to improve the efficiency and reliability of railway maintenance operations. This thesis explores the potential of ML algorithms in predicting equipment failures, optimizing maintenance schedules, and reducing operational costs in railway systems.
Chapter 1 provides an introduction to the research topic, outlining the background, problem statement, objectives, limitations, scope, significance, structure of the thesis, and definition of key terms. Chapter 2 presents a comprehensive literature review on predictive maintenance in railways, applications of ML in predictive maintenance, statistical analysis techniques, sensor technologies, case studies, challenges, and opportunities in the field.
Chapter 3 details the research methodology adopted in this thesis, including research design, data collection, preprocessing, feature selection, model selection, training, evaluation, validation, testing, and ethical considerations. Chapter 4 discusses the findings of the research, including predictive maintenance models, integration with railway maintenance systems, performance evaluation, comparisons with traditional approaches, case studies, implementation challenges, cost-benefit analysis, and future research directions.
Finally, Chapter 5 provides a conclusion and summary of the thesis, summarizing the findings, contributions to the field, implications for practice, recommendations for future work, and a concluding remark. This thesis aims to contribute to the growing body of knowledge on ML for predictive maintenance in railways and provide insights for industry practitioners, researchers, and policymakers in the field.
[ad_2]
Purchase Detail
Download the complete project materials to this project with Abstract, Chapters 1 – 5, References and Appendix (Questionaire, Charts, etc), Click Here to place an order via whatsapp. Got question or enquiry; Click here to chat us up via Whatsapp.
You can also call 08111770269 or +2348059541956 to place an order or use the whatsapp button below to chat us up.
Bank details are stated below.
Bank: UBA
Account No: 1021412898
Account Name: Starnet Innovations Limited
The Blazingprojects Mobile App
Download and install the Blazingprojects Mobile App from Google Play to enjoy over 50,000 project topics and materials from 73 departments, completely offline (no internet needed) with monthly update to topics, click here to install.